Large Pretrained AI: Multimodal Knowledge Graph and Biological Language Models
The widespread use of AI in medicine and science requires us to incorporate increasingly complex descriptors, constraints, and causal structures into ML models. To achieve deeper reasoning, we must model knowledge in the form of compact mathematical statements of physical relationships, knowledge graphs, prior distributions, and other complex rationales. For example, human experts use knowledge about tasks, such as understanding the laws of chemical biology or molecular binding, to navigate these tasks seamlessly.
We develop techniques to incorporate such inductive biases (the assumptions that algorithms use to make predictions for inputs they have not encountered during training) into AI models. The resulting foundational models trained on broad data at scale can be adapted to a wide range of downstream tasks and can handle multiple modalities, such as molecular structures and biological sequences.
We are also developing techniques for self-supervised learning, particularly neural nets that can identify essential, compact features useful to solve many tasks simultaneously without relying on predefined labels. For example, pretraining large models on unlabeled data can transfer concepts learned in one domain to a different domain, even when no labels in the target domain are available. This strategy allows us to train neural nets on a large scale and to process different downstream tasks using shared parameters. These models can achieve competitive performance on pretraining tasks and perform zero-shot inference on novel tasks without introducing additional parameters.
We are looking to transfer the prediction prowess of these models from one data type to another and design contextually adaptive AI that can extrapolate to new tasks and situations, such as new molecular scaffolds, new cell types, and new clinical diseases.
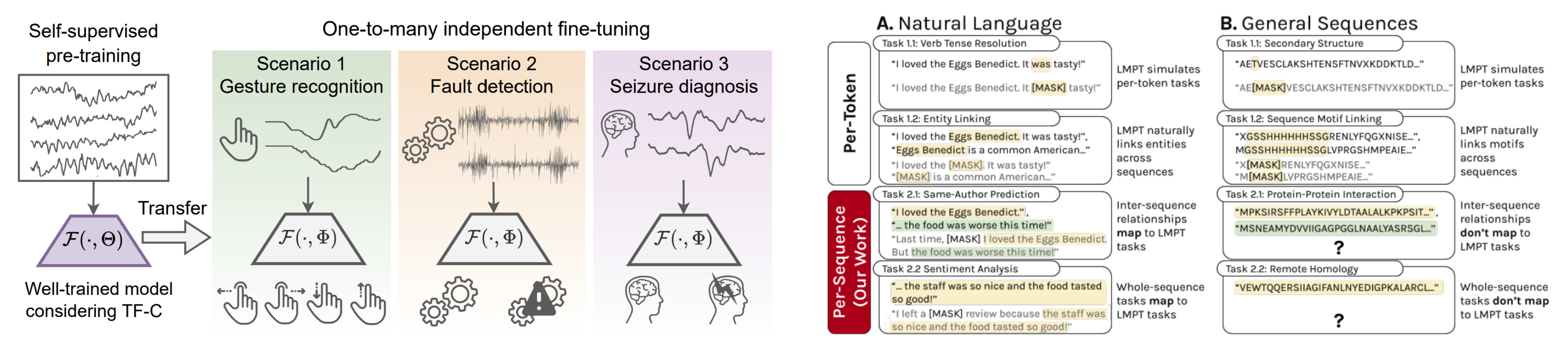
Example related publications:
Structure Inducing Pre-Training
Self-Supervised Contrastive Pre-Training For Time Series via Time-Frequency Consistency
Graph-Guided Network for Irregularly Sampled Multivariate Time Series
Graph Meta Learning via Local Subgraphs
Machine Learning for Integrating Data in Biology and Medicine: Principles, Practice, and Opportunities
Data Fusion by Matrix Factorization
Geometric Deep Learning
The critical capability expected of machine learning used in medicine and science is to incorporate structure, geometry, symmetry and be grounded in knowledge. The challenge, however, is that prevailing deep and representation learning algorithms are designed for data with a regular, grid-like structure (e.g., images have a 2D grid structure, and sequences have a linear 1D structure). As a result, these algorithms cannot truly exploit complex, interconnected data with irregular interactions between entities, i.e., edges, the essence of graphs.
We are developing methods to address these challenges. The notion of flexible representations is at the technical core of these methods. We formalize this idea by specifying deep transformation functions, or graph neural networks, that map nodes, or larger graph structures, to points in a low-dimensional space, termed embeddings. Importantly, these functions are optimized to embed the input dataset to perform algebraic operations in the learned latent spaces reflecting the dataset’s structure and topology.
Our research has been at the forefront of geometric deep learning research and pioneered graph neural networks (GNNs) for biology and medicine. This allows us to apply deep learning much more broadly and set sights on new frontiers beyond classic applications of deep learning in computer vision and natural language processing.
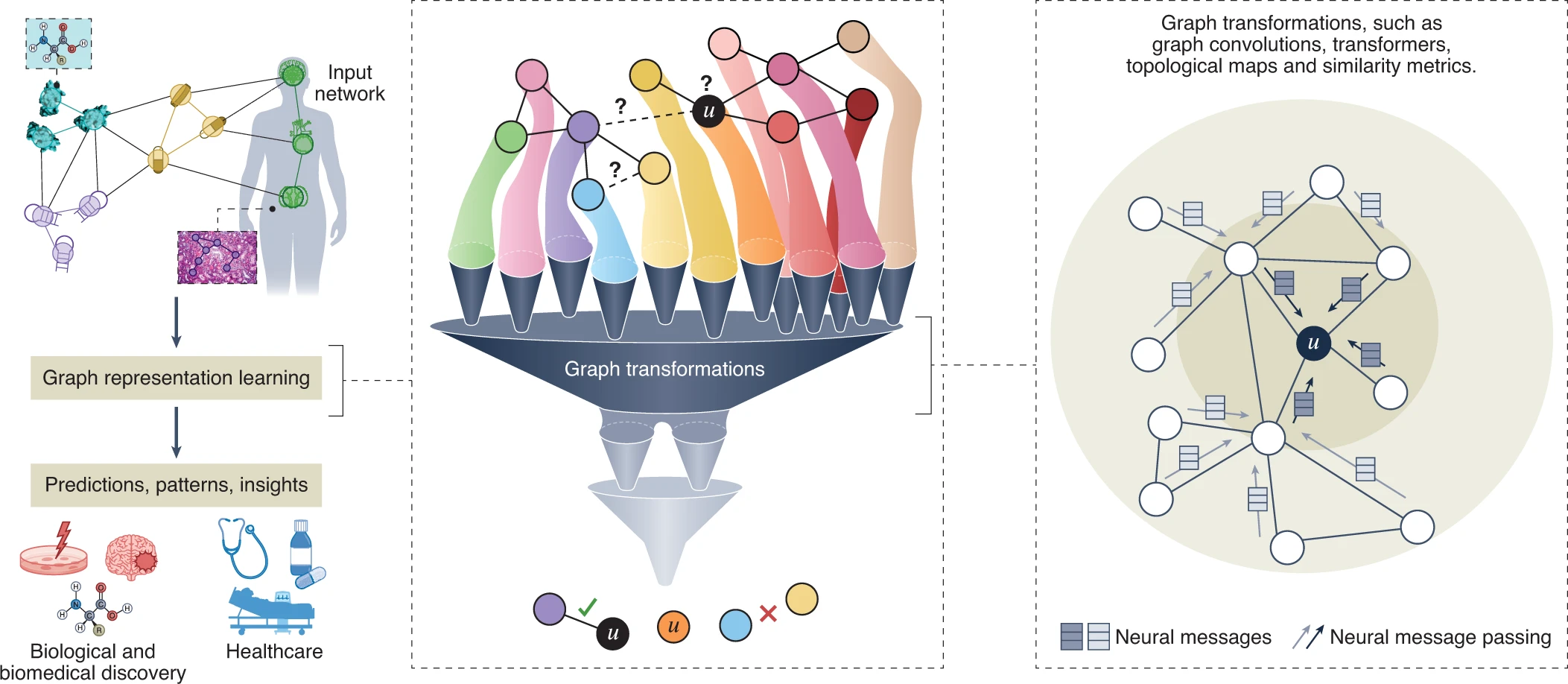
Perspective
Graph Representation Learning for Biomedicine and Healthcare in Nature Biomedical Engineering
Example related publications:
Towards a Unified Framework for Fair and Stable Graph Representation Learning
Multimodal Learning with Graphs
Probing GNN Explainers: A Rigorous Theoretical and Empirical Analysis of GNN Explanation Methods
GNN Explainer: Generating Explanations for Graph Neural Networks
Embedding Logical Queries on Knowledge Graphs
Pre-Training Graph Neural Networks
Subgraph Neural Networks
Learning Structural Node Embeddings via Diffusion Wavelets
Modeling Polypharmacy Side Effects with Graph Convolutional Networks
GNNGuard: Defending Graph Neural Networks against Adversarial Attacks
Podcasts:
MITxHarvard Women in Artificial Intelligence [YouTube Interview]
Educational resources:
Slides: Graph Neural Networks in Computational Biology
Recording: Graph Neural Networks in Computational Biology
Towards Precision Medicine with Graph Representation Learning
Multi-Scale Individualized, Contextualized, and Network Learning Systems
Networks, or graphs, pervade biomedical data — from the molecular level to the level of connections between diseases in a person and the societal level encompassing all human interactions. These interactions at different levels give rise to a bewildering degree of complexity which is only likely to be fully understood through a holistic and integrated systems view and the study of combined, multi-level networks.
We focus on designing algorithmic solutions to optimize and manipulate networked systems for useful purposes and to predict their behavior, such as how genomics influences human traits in a particular environment. We also actively develop methods to harness rich interaction data and network dynamics. We scale up the analyses to see structure in massive amounts of data that are too complex to be detected with other methods.
Many diseases are controlled by more than one gene with very different patterns of mutations across different cells within the same disease. A powerful way to interpret these genetic events is to organize them into interactomes — networks of genes that participate in dysregulated processes. Disruptions of normal gene behavior may be infrequent at the nucleotide or gene level but can be substantially more common when considering impacts in a larger biological process. Understanding the disease in this way suggests that effective therapies might need to target biological systems — interactomes — larger than those encoded by single genes to successfully intervene against the disease.
In our work, we develop geometric machine learning methods to study the impact of drugs on proteins perturbed in disease. Among others, we used the methods to predict drugs effective for coronavirus infections. We showed that 76 of the 77 predicted drugs that reduced viral infection in human cells do not bind the proteins targeted by the virus, indicating that these “network drugs” rely on network mechanisms, targeting proteins near the viral module.
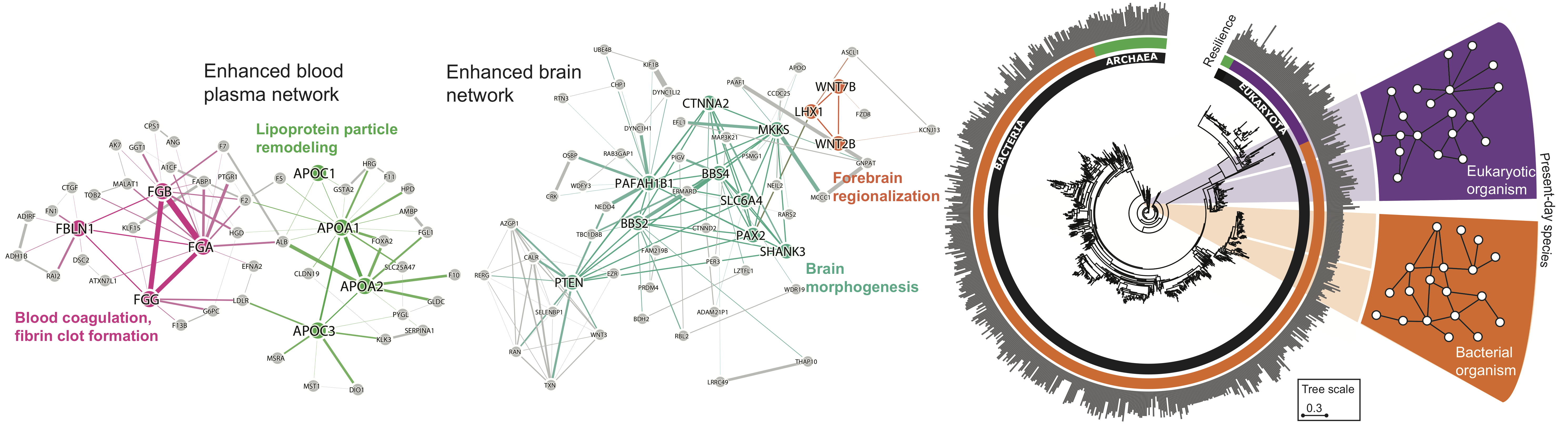
Example related publications:
Deep Learning for Diagnosing Patients with Rare Genetic Diseases
Evolution of Resilience in Protein Interactomes Across the Tree of Life
Identification of Disease Treatment Mechanisms through the Multiscale Interactome
Network Enhancement as a General Method to Denoise Weighted Biological Networks
Discovering Novel Cell Types Across Heterogeneous Single-Cell Experiments
Mutual Interactors as a Principle for Phenotype Discovery in Molecular Interaction Networks
Predicting Multicellular Function Through Multi-Layer Tissue Networks
Sparse Dictionary Learning Recovers Pleiotropy From Human Cell Fitness Screens
Better Drug Design, Molecule Optimization, High-Throughput Perturbations, Interaction Screening
For centuries, the scientific method — the fundamental practice of science that scientists use to explain the natural world systematically and logically — has remained essentially the same. We have already made substantial progress in using machine learning to change that. Within the context of drug development, we develop methods that accelerate the discovery of safer and more effective medicines and advance therapeutic science. We ask fundamental questions about machine learning methods needed to advance therapeutic science, and, critically, also conduct in-depth work that integrates algorithmic advances with experimental collaborators.
The study of how two or more molecular structures (e.g., drug and enzyme or protein) fit together is typically accomplished by either molecular docking or perturbation-response and chemo-genetic profiling, both of which are beginning to harness the power of existing machine learning. At this moment, however, molecular modeling is conducted in a highly constrained design space, owing to the lack of interactomes in different cell types and the limited power to explore larger design spaces. We study the role of cellular and tissue contexts in interactomes, particularly those affected by the disease, and design machine learning model to help design chemical and genetic perturbations that reverse disease effects. We develop predictive and generative AI models that produce interpretable outputs and actionable hypotheses to maximize the yield of downstream experiments in the lab.
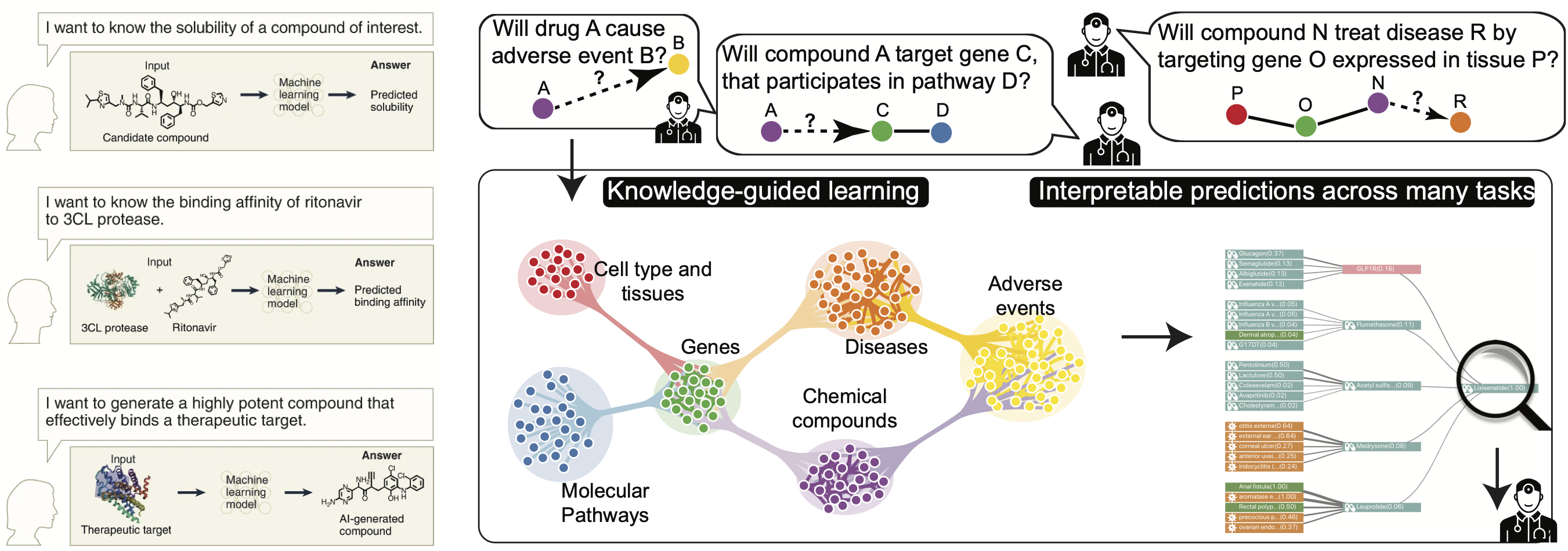
Example related publications:
Zero-Shot Prediction of Therapeutic Use With Geometric Deep Learning and Clinician Centered Design
Network Medicine Framework for Identifying Drug Repurposing Opportunities for COVID-19
Modeling Polypharmacy Side Effects with Graph Convolutional Networks
Prioritizing Network Communities
Extending the Nested Model for User-Centric XAI: A Design Study on GNN-based Drug Repurposing
DeepPurpose: A Deep Learning Library for Drug-Target Interaction Prediction
Population-Scale Identification of Differential Adverse Events Before and During a Pandemic
Gene Prioritization by Compressive Data Fusion and Chaining
A Comprehensive Structural, Biochemical and Biological Profiling of the Human NUDIX Hydrolase Family
Podcasts:
Bayer Foundation Report (Bayer’s Early Excellence in Science Award)
Machine Learning for Drug Development
FutureDose Tech
MIT AI Cures Blog
Initiatives:
Robust Foundation, Modern Data Management, Scalable Infrastructure for Discovery in the Age of AI
Faced with skyrocketing costs and high failure rates, researchers are looking at ways to make drug discovery and development more efficient through automation, AI and new data modalities. AI has become woven into therapeutic discovery since the emergence of deep learning. To support the adoption of AI in therapeutic science, we need a composable machine learning foundation spanning the stages of drug discovery that can help implement AI methods most suitable for drug discovery applications.
Although biological and chemical research generates a wealth of data, most generated datasets are not readily suitable for AI analyses because they are incomplete.
-
First, the lack of AI-ready datasets and standardized knowledge representations prevent scientists from formulating relevant questions in drug discovery as solvable AI tasks — posing the challenge of how to link scientific workflows, protocols and other information into computable knowledge.
-
Second, datasets can be multimodal and of many different types, including experimental readouts, curated annotations and metadata, and are scattered around biochemical repositories — posing the challenge of how to collect and annotate datasets to establish best practices for AI analysis, as poor understanding of the data can lead to misinterpreted results and misused methods.
-
Finally, despite the promising in silico performance of AI methods, their use in practice, such as for rare diseases and novel drugs in development, has been limited — posing the challenge of how to assess methodological advances in a manner that allows robust and transparent comparison and represents what one would expect in a real-world implementation.
To this end, AI methods and datasets must be integrated, and data stewardship strategies must be developed to reduce data-processing and data-sharing burdens. This includes optimally balanced and algorithmically sound approaches to ensure that biochemical information (including genomic data) is findable, accessible, interoperable and reusable10, as well as engaging communities in determining what data are needed.
We founded Therapeutics Data Commons (TDC), an initiative to access and evaluate AI capability across therapeutic modalities and stages of discovery, establishing a foundation for understanding which AI methods are most suitable for advancing therapeutic science and why. At its core, the Commons is a collection of AI-solvable tasks, AI-ready datasets, and curated benchmarks that cover a wide range of therapeutic products (AI tasks for small molecules, including drug response and synergy prediction, AI tasks for macromolecules, including paratope and epitope prediction; and AI tasks for cell and gene therapies, including CRISPR repair prediction) across all stages of discovery (target-discovery tasks, such as identification of disease-associated therapeutic targets; activity-modeling tasks, such as quantum-mechanical energy prediction; drug efficacy and safety tasks, such as molecule generation; and manufacturing tasks, such as yield outcome prediction).
Achieving broad use of AI in therapeutic science requires coordinated community initiatives that earn the trust of diverse groups of scientists. The Commons creates a meeting point between biochemical and AI scientists. This makes it possible to look at AI from different perspectives and with a wide variety of mindsets across traditional boundaries and multiple disciplines. We envision that the Commons can considerably accelerate machine learning model development, validation, and transition into biomedical and clinical implementation.
Perspectives:
Artificial Intelligence Foundation for Therapeutic Science in Nature Chemical Biology
Example related publications:
Therapeutics Data Commons: Machine Learning Datasets and Tasks for Therapeutics [Slides]
Building a Knowledge Graph to Enable Precision Medicine
Evaluating Explainability for Graph Neural Networks
NIMFA: A Python Library for Non-negative Matrix Factorization