Relational data in biological systems – such as the cellular interactome, single cell similarity graphs, gene co-expression networks, and patient interaction networks – can be represented by graph structures. Biological networks are often comprised of diverse data modalities; thus, they are poorly modeled by homogenously typed networks. Instead, interconnected objects from various modalities can be represented as a single multigraph with heterogeneous knowledge-informed node and edge types. We develop metapaths, an R software package to implement meta paths and perform meta path-based similarity search in biological knowledge graphs.
Meta paths are a general graph-theoretic approach for flexible similarity search in large networks. While they are widely used in biomedical network analysis, there is currently no package available in R that would offer a wide range of support for meta paths.
Meta paths are sequences of node types that define a walk from the origin node to the destination node. Informative metapaths in knowledge graphs (KGs) are often engineered by hand based on domain knowledge or expertise (e.g., the meta path DRS is clinically meaningful, since it describes associations between a disease and the side effects of its treatments, whereas the meta path PSF would not be). Alternatively, optimal meta paths can be discovered in an unsupervised fashion by feature selection metrics (e.g., maximal spanning tree, Laplacian score, or ranking based on meta path frequency or uniqueness), among other approaches. Once informative meta paths for a given KG have been defined, these meta paths define the semantics of the relationships between nodes in the KG, enabling down-stream machine learning analyses such as link prediction, node classification, and subgraph prediction.
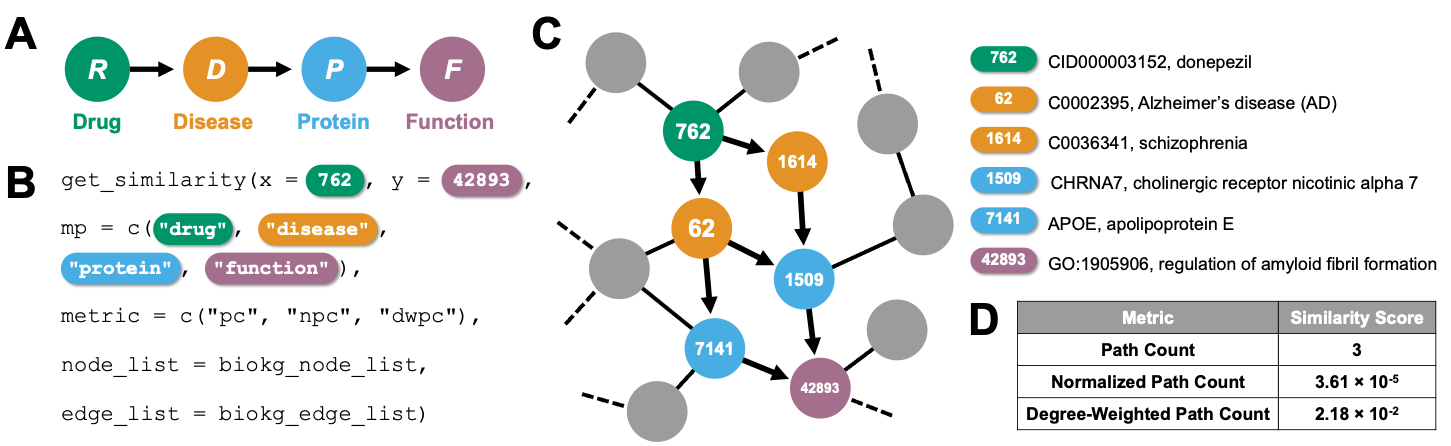
Although various algorithms exist to model meta path-based node simi-larities in a KG, a unifying framework is lacking to compute and compare these similarity scores. We introduce metapaths/ which introduces meta paths in the R ecosystem. The metapaths package enables the computation of meta-path-based similarity search in heterogeneous KGs.
Publication
metapaths: similarity search in heterogene-ous knowledge graphs via meta paths
Ayush Noori, Amelia L.M. Tan, Michelle M. Li, and Marinka Zitnik
Bioinformatics 2023
@article{noori2023metapaths,
title={metapaths: similarity search in heterogeneous knowledge graphs via meta paths},
author={Noori, Ayush and Li, Michelle M and Tan, Amelia LM and Zitnik, Marinka},
journal={Bioinformatics},
pages={btad297},
year={2023},
publisher={Oxford University Press}
}
Code
Implementation in R together with documentation and examples of usage is available in the GitHub repository.