Foundation models, especially LLMs, are profoundly transforming deep learning. Instead of training many task-specific models, we can adapt a single pretrained model to many tasks via few-shot prompting or fine-tuning. However, current foundation models apply to sequence data but not to time series, which present unique challenges due to the inherent diverse and multi-domain time series datasets, diverging task specifications across forecasting, classification and other types of tasks, and the apparent need for task-specialized models.
We developed UniTS, a unified time series model that supports a universal task specification, accommodating classification, forecasting, imputation, and anomaly detection tasks. This is achieved through a novel unified network backbone, which incorporates sequence and variable attention along with a dynamic linear operator and is trained as a unified model.
Across 38 multi-domain datasets, UniTS demonstrates superior performance compared to task-specific models and repurposed natural language-based LLMs. UniTS exhibits remarkable zero-shot, few-shot, and prompt learning capabilities when evaluated on new data domains and tasks. We will release the source code and datasets.
The machine learning community has long pursued the development of unified models capable of handling multiple tasks. Such unified and general-purpose models have been developed for language and vision, where a single pretrained foundation model can be adapted to new tasks with little or no additional training via multi-task learning, few-shot learning, zero-shot learning, and prompt learning.
However, general-purpose models for time series have been relatively unexplored. Time series datasets are abundant across many domains—including medicine, engineering, and science—and are used for a broad range of tasks such as forecasting, classification, imputation, and anomaly detection. Current time series models, however, require either fine-tuning or the specification of new task- and dataset-specific modules to transfer to new datasets and tasks, which can lead to overfitting, hinder few- or zero-shot transfer, and burden users.
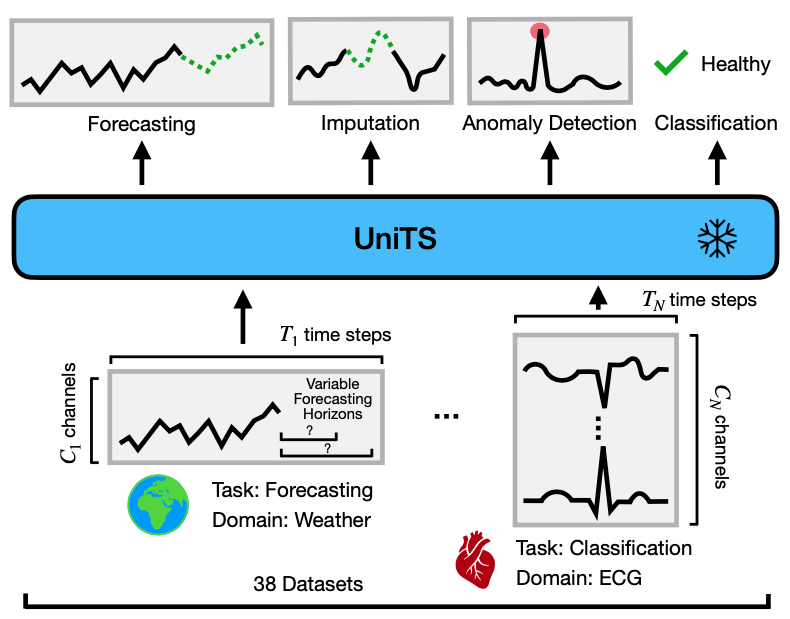
Building a unified time series model presents unique challenges:
-
Multi-domain temporal dynamics: Unified models learn general knowledge by co-training on diverse data sources, but time series data present wide variability in temporal dynamics across domains. Further, time series data may have heterogeneous data representations such as the number of variables, the definition of sensors, and length of observations. Such heterogeneity in time series data hinders the use of unified models developed for other domains. Therefore, a unified model must be designed and trained to capture general temporal dynamics that transfer to new downstream datasets, regardless of data representation.
-
Diverging task specifications: Common tasks on time series data have fundamentally different objectives. For example, forecasting entails predicting future values in a time series, akin to a regression problem, while classification is a discrete decision-making process made on an entire sample. Further, the same task across different datasets may require different specifications, such as generative tasks that vary in length and recognition tasks featuring multiple categories. Existing time series models define task-specific modules to handle each task, which compromises their adaptability to diverse types of tasks. A unified model must be able to adapt to changing task specifications from users.
-
Requirement for task-specific time series modules: Unified models employ shared weights across various tasks, enhancing their generalization ability. owever, the distinct task-specific modules for each dataset in previous approaches require the fine-tuning of these modules. This process often demands finely adjusted training parameters as well as a moderate dataset size per task, hindering rapid adaptation to new tasks. Such a strategy contradicts the concept of a unified model designed to manage multiple tasks concurrently.
Overview of UniTS
UniTS is a unified time series model that processes various tasks with shared parameters without resorting to any task-specific modules. UniTS achieves competitive performance in trained tasks and can perform zero-shot inference on novel tasks without the need for additional parameters. UniTS makes the following novel contributions:
1) Universal task specification with prompting: UniTS uses a prompting-based framework to convert various tasks into a unified token representation, creating a universal specification for all tasks.
2) Data-domain agnostic network: UniTS employs self-attention across both sequence and variable dimensions to accommodate diverse data shapes. We introduce a dynamic linear operator to model dense relations between data points in sequences of any length. As a result, UniTS can process multi-domain time series with diverse variables and lengths without the need to modify the network structure.
3) Unified model with fully shared weights: Leveraging the universal task specification and data-domain agnostic network, UniTS has shared weights across tasks. To improve UniTS’s generalization ability, a unified masked reconstruction pretraining scheme is introduced to handle both generative and recognition tasks within a unified model.
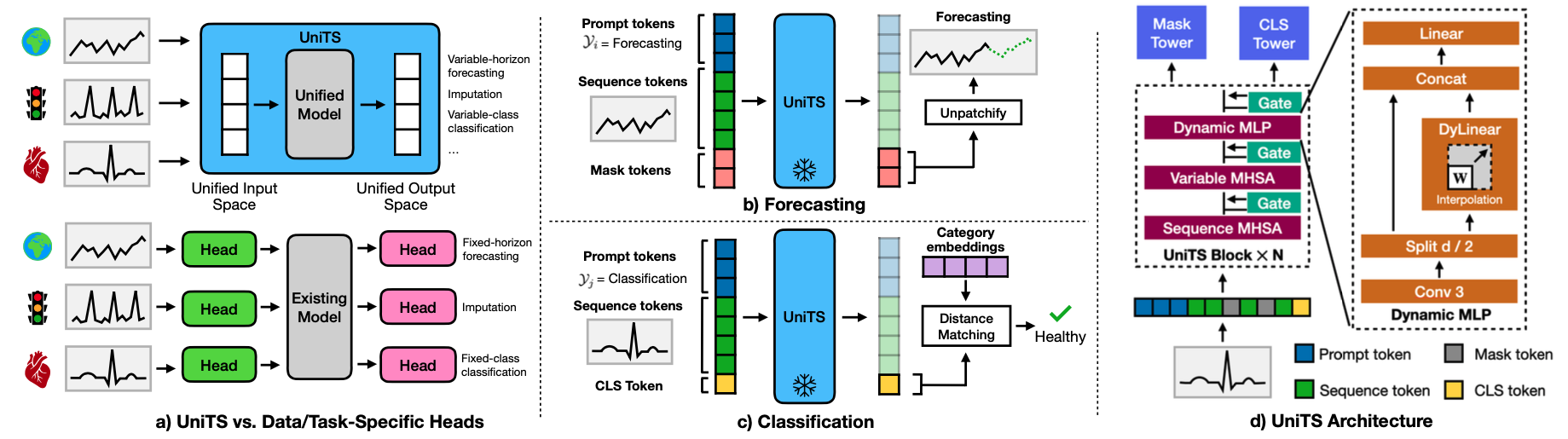
In a challenging multi-domain/task setting, a single UniTS with fully shared weights successfully handles 38 diverse tasks, indicating its potential as a unified time series model. UniTS outperforms top-performing baselines, which require data and task-specific modules, by achieving the highest average performance and the best results on 27 out of the total 38 tasks.
Additionally, UniTS can perform zero-shot and prompt-based learning. It excels in zero-shot forecasting for out-of-domain data, handling new forecasting horizons and numbers of variables/sensors. For instance, in one-step forecasting with new lengths, UniTS outperforms the top baseline model, which relies on sliding windows, by 10.5%. In the prompt learning regime, a fixed, self-supervised pretrained UniTS is adapted to new tasks, achieving performance comparable to UniTS’s supervised counterpart. In 20 forecasting datasets, prompted UniTS outperforms the supervised version, improving the MAE from 0.381 to 0.376.
UniTS demonstrates exceptional performance in few-shot transfer learning, effectively handling tasks such as imputation, anomaly detection, and out-of-domain forecasting and classification without requiring specialized data or task-specific modules. For instance, UniTS outperforms the strongest baseline by 12.4% (MSE) on imputation tasks and 2.3% (F1-score) on anomaly detection tasks.
UniTS shows the potential of unified models for time series and paves the way for generalist models in time series analysis.
Publication
UniTS: Building a Unified Time Series Model
Shanghua Gao, Teddy Koker, Owen Queen, Thomas Hartvigsen, Theodoros Tsiligkaridis, and Marinka Zitnik
In Review 2024 [arXiv]
@article{gao2024building,
title={UniTS: Building a Unified Time Series Model},
author={Gao, Shanghua and Koker, Teddy and Queen, Owen and Hartvigsen, Thomas and Tsiligkaridis, Theodoros and Zitnik, Marinka},
journal={arXiv},
url={https://arxiv.org/abs/2403.00131},
year={2024}
}
Code Availability
Pytorch implementation of UniTS is available in the GitHub repository.