Of the several thousand diseases that affect humans, only about 500 have treatments approved by the U.S. Food and Drug Administration. Even for those with approved treatments, discovering new drugs can offer alternative options that cause fewer side effects and replace drugs that are ineffective for certain patient groups. However, identifying new therapeutic opportunities for diseases with limited treatment options remains a challenge, as existing algorithms often perform poorly.
Here, we leverage recent advances in geometric deep learning and human-centered AI to introduce TxGNN, a model for identifying therapeutic opportunities for diseases with limited treatment options and minimal molecular understanding. TxGNN is a graph neural network pre-trained on a comprehensive knowledge graph of 17,080 clinically-recognized diseases and 7,957 therapeutic candidates. The model can process various therapeutic tasks, such as indication and contraindication prediction, in a unified formulation. Once trained, we show that TxGNN can perform zero-shot inference on new diseases without additional parameters or fine-tuning on ground truth labels.
Evaluation of TxGNN shows significant improvements over existing methods, with up to 49.2% higher accuracy in indication tasks and 35.1% higher accuracy in contraindication tasks. TxGNN can also predict therapeutic use for new drugs developed since June 2021. To facilitate interpretation and analysis of the model's predictions by clinicians, we develop a human-AI explorer for TxGNN and evaluate its usability with medical experts. Finally, we demonstrate that TxGNN's novel predictions are consistent with off-label prescription decisions made by clinicians in a large healthcare system.
These label-efficient and clinician-centered learning systems pave the way for improvements for many therapeutic tasks.
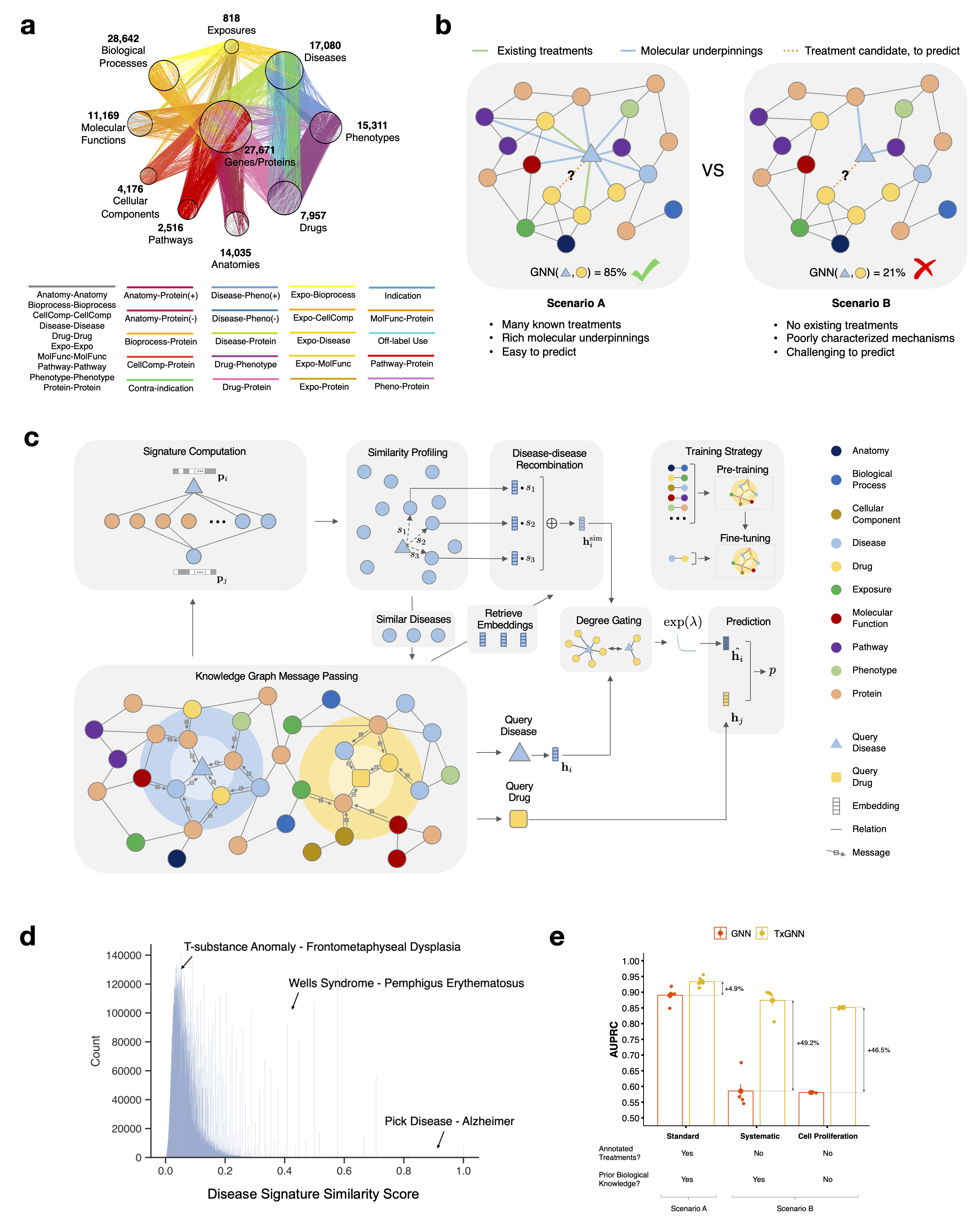
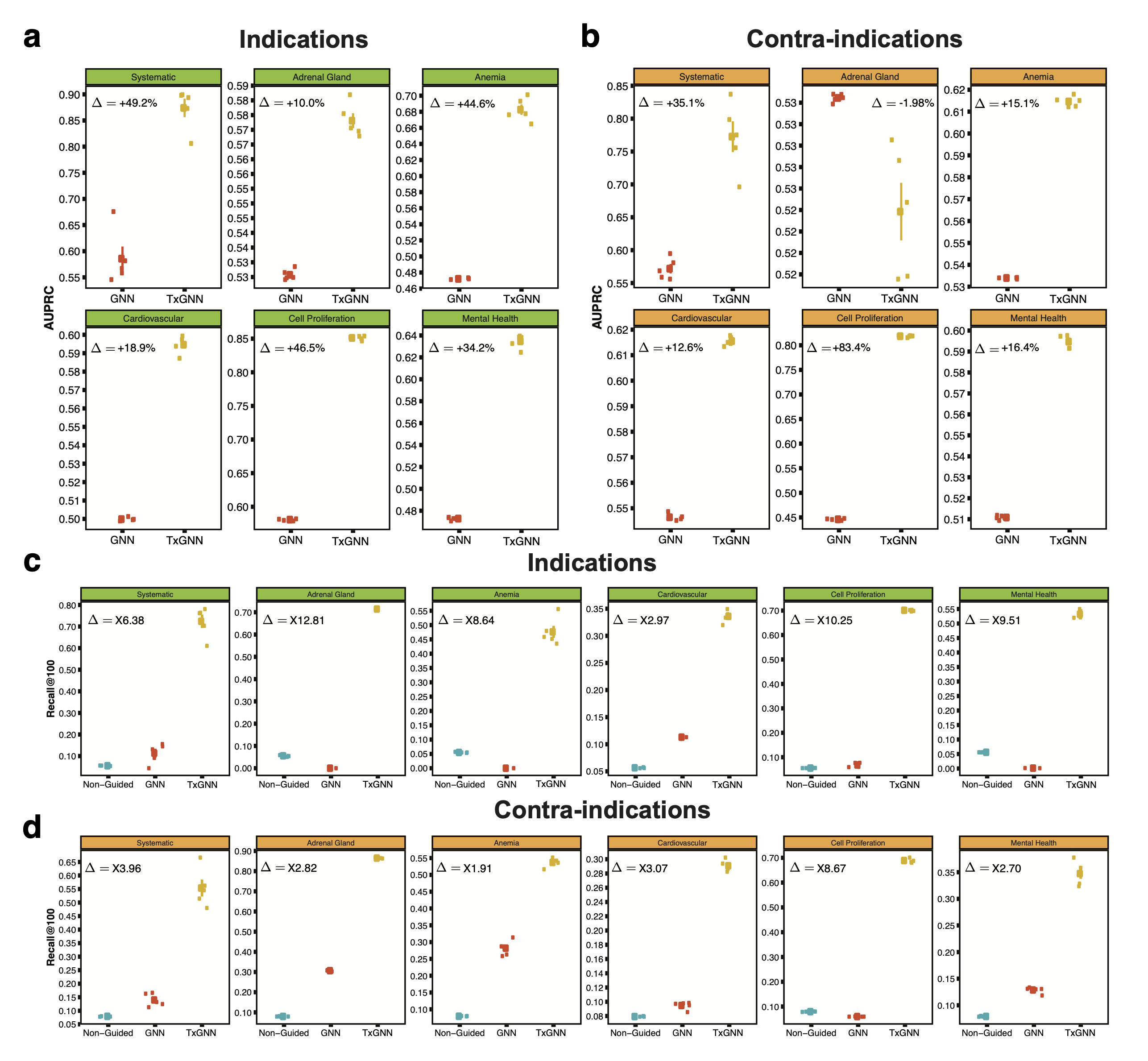
Publication
Zero-shot Prediction of Therapeutic Use with Geometric Deep Learning and Clinician Centered Design
Kexin Huang*, Payal Chandak*, Qianwen Wang, Shreyas Havaldar, Akhil Vaid, Jure Leskovec, Girish Nadkarni, Benjamin S. Glicksberg, Nils Gehlenborg and Marinka Zitnik
In Review 2023 [medRxiv]
@article{huang2023zeroshot,
title={Zero-shot Prediction of Therapeutic Use with Geometric Deep Learning and Clinician Centered Design},
author={Huang, Kexin and Chandak, Payal and Wang, Qianwen and Havaldar, Shreyas and Vaid, Akhil and Leskovec, Jure and Nadkarni, Girish and Glicksberg, Benjamin and Gehlenborg, Nils and Zitnik, Marinka},
journal = {medRxiv},
doi = {10.1101/2023.03.19.23287458},
volume={},
number={},
pages={},
year={2023},
publisher={}
}
Code
PyTorch implementation together with documentation and examples of usage is available in the GitHub repository.
Human-AI Explorer for TxGNN
To facilitate interpretation and analysis of the model’s predictions by clinicians, we develop a human-AI explorer for TxGNN and evaluate its usability with medical experts. The TxGNN explorer is available at http://txgnn.org.