Deep learning has made rapid advances in modeling molecular sequencing data. Despite achieving high performance on benchmarks, it remains unclear to what extent deep learning models learn general principles and generalize to previously unseen sequences.
Benchmarks traditionally interrogate model generalizability by generating metadata based (MB) or sequence-similarity based (SB) train and test splits of input data before assessing model performance. Here, we show that this approach mischaracterizes model generalizability by failing to consider the full spectrum of cross-split overlap, i.e., similarity between train and test splits.
We introduce SPECTRA, a spectral framework for comprehensive model evaluation. For a given model and input data, SPECTRA plots model performance as a function of decreasing cross-split overlap and reports the area under this curve as a measure of generalizability.
We use SPECTRA with 18 sequencing datasets and phenotypes ranging from antibiotic resistance in tuberculosis to protein-ligand binding to evaluate the generalizability of 19 state-of-the-art deep learning models, including large language models, graph neural networks, diffusion models, and convolutional neural networks. We show that SB and MB splits provide an incomplete assessment of model generalizability.
Using SPECTRA, we find as cross-split overlap decreases, deep learning models consistently exhibit a reduction in performance in a task- and model-dependent manner. Although no model consistently achieved the highest performance across all tasks, we show that deep learning models can, in some cases, generalize to previously unseen sequences on specific tasks. SPECTRA paves the way toward a better understanding of how foundation models generalize in biology.
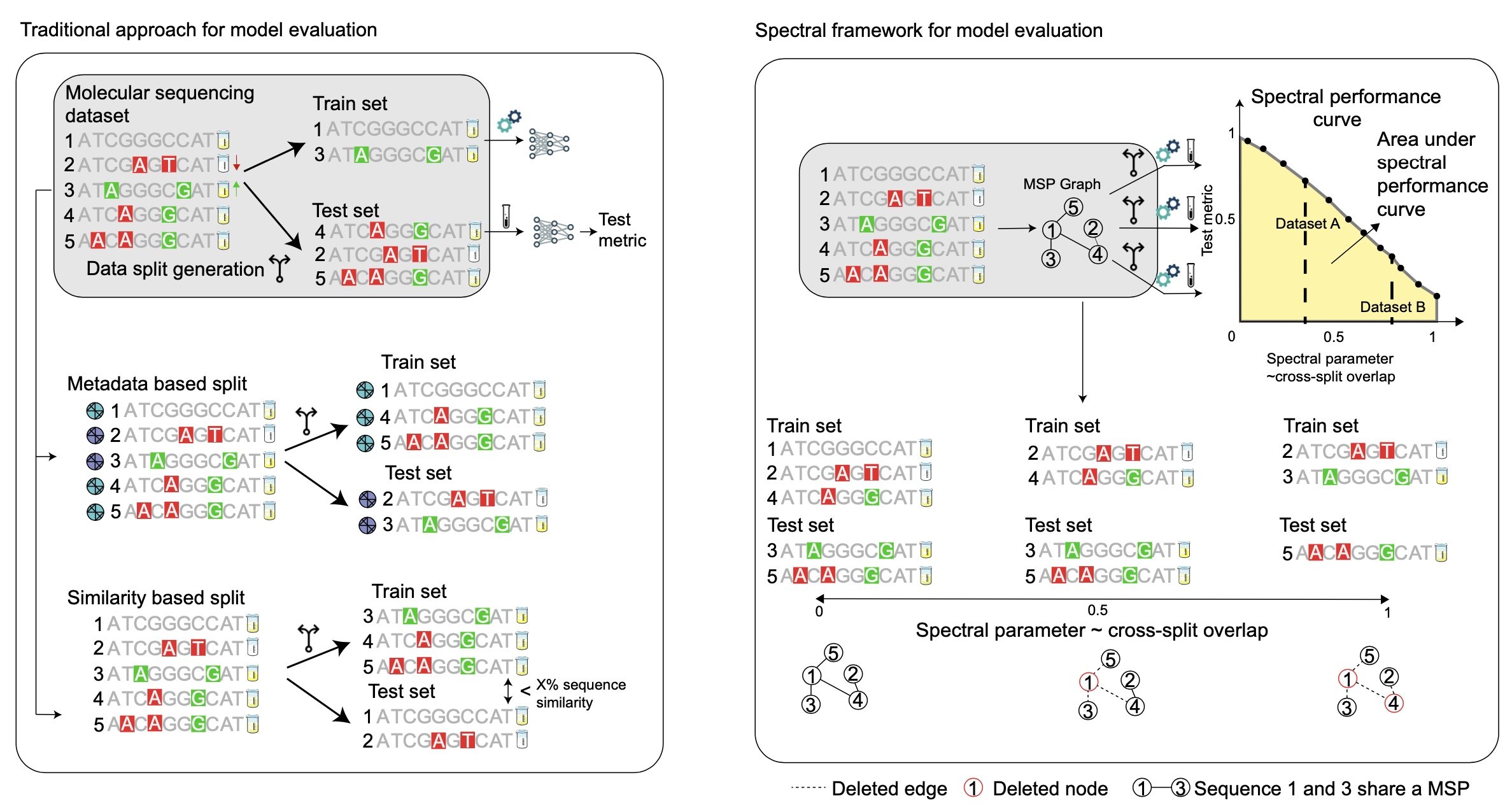
Publication
Evaluating Generalizability of Artificial Intelligence Models for Molecular Datasets
Yasha Ektefaie, Andrew Shen, Daria Bykova, Maximillian Marin, Marinka Zitnik* and Maha Farhat*
In Review 2024 [bioRxiv]
@article{ektefaie2024evaluating,
title={Evaluating Generalizability of Artificial Intelligence Models for Molecular Datasets},
author={Ektefaie, Yasha and Shen, Andrew and Bykova, Daria and Maximillian, Marin and Zitnik, Marinka* and Farhat, Maha*},
journal={bioRxiv},
url={https://www.biorxiv.org/content/10.1101/2024.02.25.581982v1},
year={2024}
}
Code Availability
Pytorch implementation of SPECTRA is available in the GitHub repository.