Language model pre-training and derived methods are incredibly impactful in machine learning. However, there remains considerable uncertainty on exactly why pre-training helps improve performance for fine-tuning tasks. This is especially true when attempting to adapt language-model pre-training to domains outside of natural language. We analyze this problem by exploring how existing pre-training methods impose relational structure in their induced per-sample latent spaces—i.e., what constraints do pre-training methods impose on the distance or geometry between the pre-trained embeddings of two samples xi and xj.
Through a comprehensive review of existing pre-training methods, we find that this question remains open. This is true despite theoretical analyses demonstrating the importance of understanding this form of induced structure. Based on this review, we introduce a descriptive framework for pre-training that allows for a granular, comprehensive understanding of how relational structure can be induced.
We present a theoretical analysis of this framework from the first principles and establish a connection between the relational inductive bias of pre-training and fine-tuning performance. We also show how to use the framework to define new pre-training methods. Finally, we build upon these findings with empirical studies on benchmarks spanning 3 data modalities and ten fine-tuning tasks. These experiments validate our theoretical analyses, inform the design of novel pre-training methods, and establish consistent improvements over a compelling suite of baseline methods.
Existing Pre-training (PT) Methods
Our review summarized 71 existing natural language processing (NLP) and NLP-derived PT methods, which are categorized into clusters based on how they impose structural constraints over the PT per sample) latent space. Clusters are arranged on axes via manual judgments on whether the imposed constraint is shallow vs. deep and implicit vs. explicit. Clusters are sized such that the area corresponds to the number of citations methods included in that cluster have received on average per month since first publication, according to Google Scholar’s citation count. In the following figure, “None” captures models that leverage no pre-training loss over the per-sample embedding. “NSP” refers to “Next sentence Prediction,” the per-sample PT task introduced in BERT. “SOP” refers to “Sentence-order Prediction,” the per-sample PT task introduced in ALBERT. Note that over 90 studies in total were considered in our review, but only 71 met the inclusion criteria to be included in this figure. These methods are described in more detail in the manuscript.
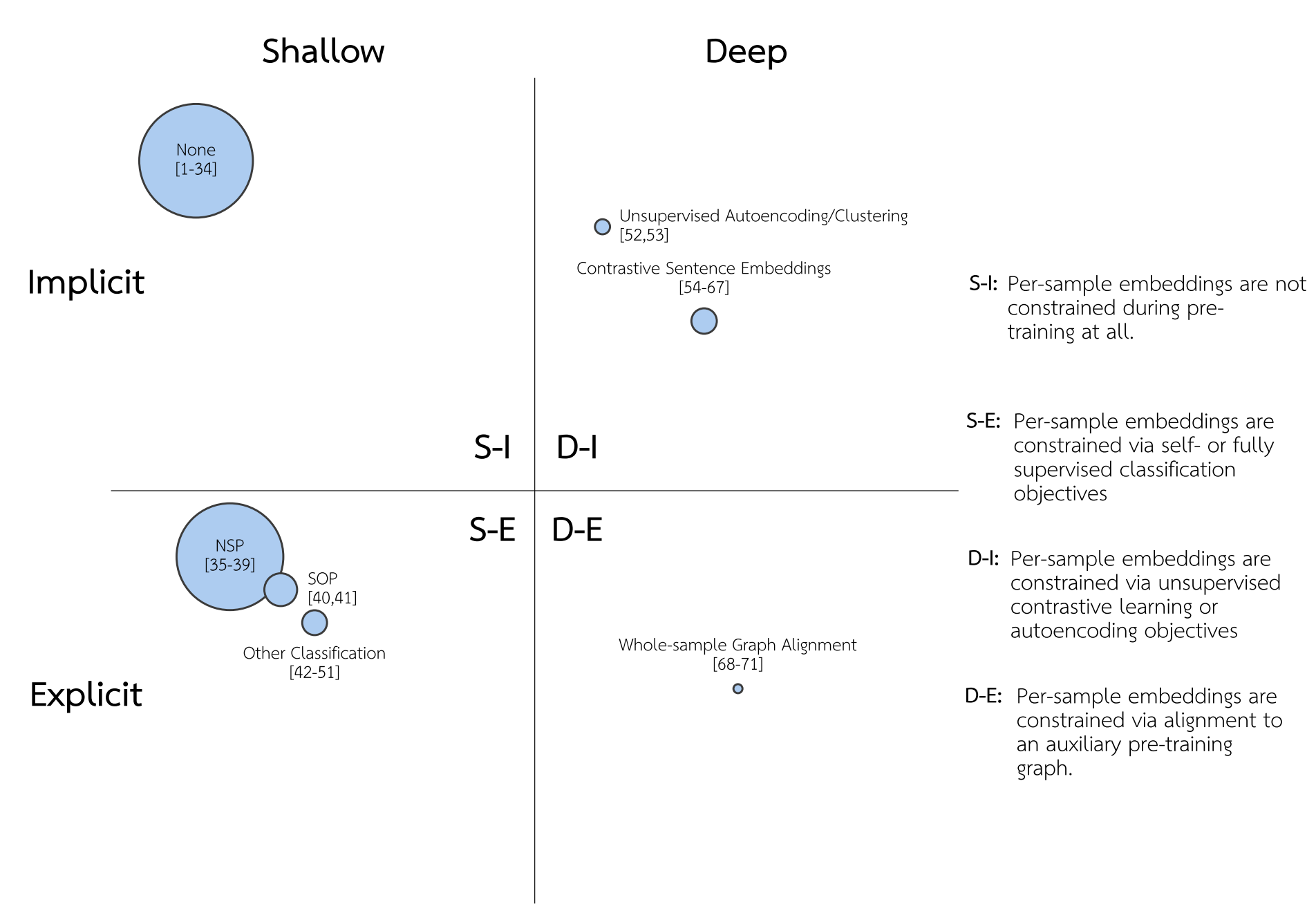
Per-sample vs. Per-token Latent Space
Language model pre-training methods produce both per-sample and per-token latent spaces. Illustrated below via the RoBERTa model are traditional language modeling objectives, which use only a masked language model loss during pre-training) only constrain the per-token latent space.
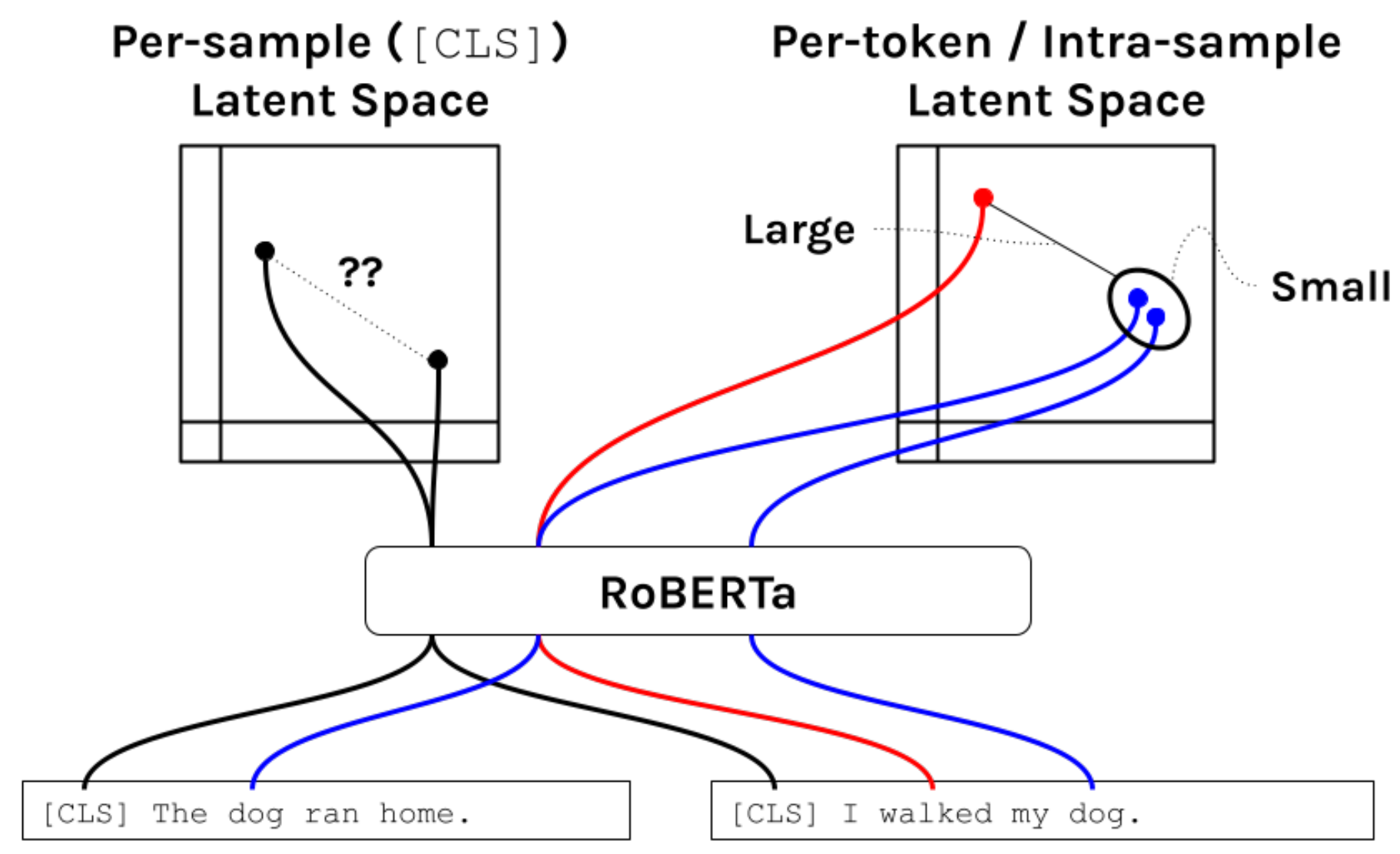
Structure Inducing Pre-Training
We re-cast the PT formulation by taking a pre-training graph GPT as an auxiliary input. GPT is used to define a new structure-inducing objective LSI, which pushes a pre-training encoder fθ to embed samples such that samples are close in the latent space if and only if they are linked in GPT.
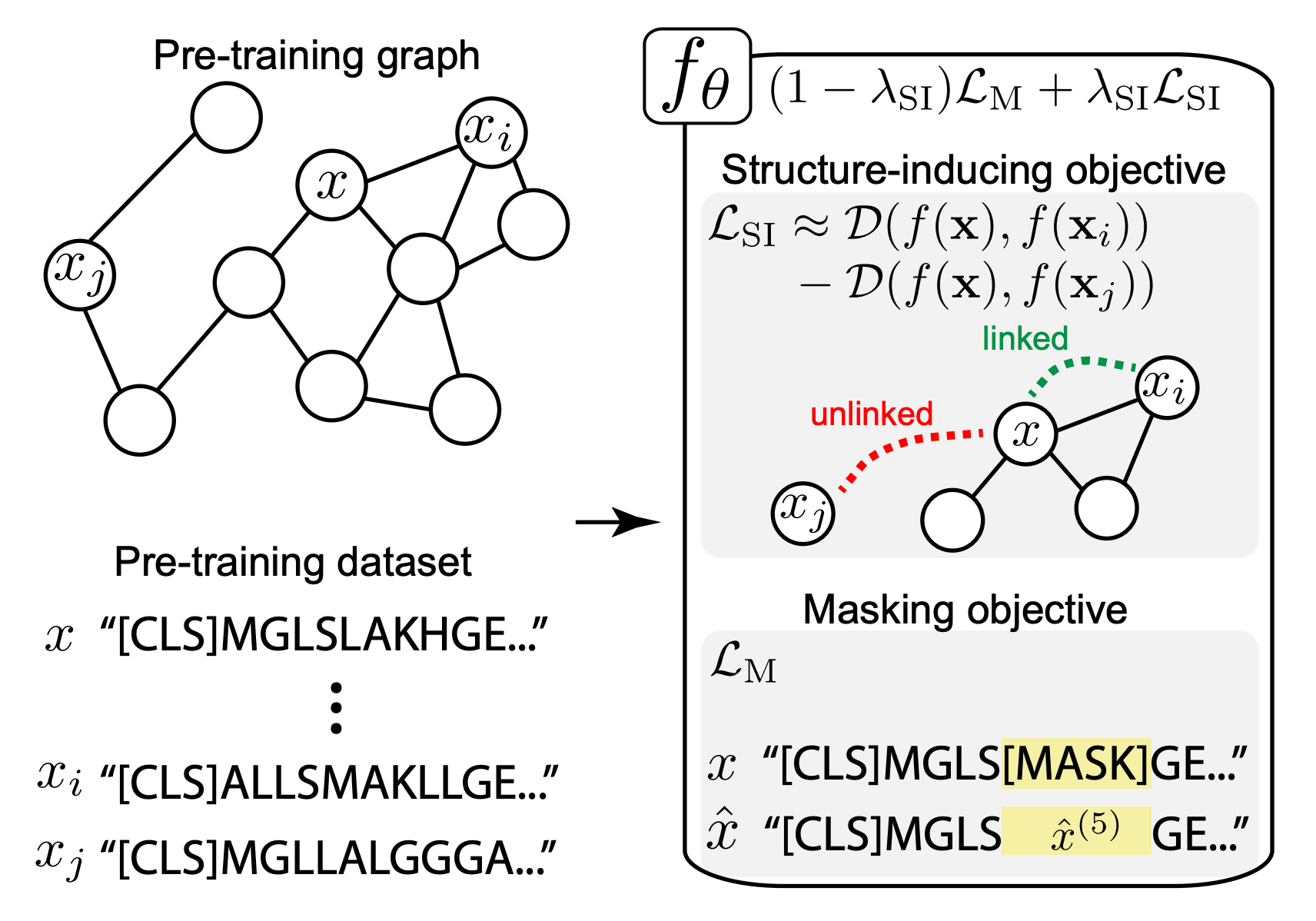
Publication
Structure Inducing Pre-Training
Matthew B.A. McDermott, Brendan Yap, Peter Szolovits and Marinka Zitnik
Nature Machine Intelligence 2023 [arXiv]
@article{mcdermott2023structure,
title={Structure Inducing Pre-Training},
author={McDermott, Matthew and Yap, Brendan and Szolovits, Peter and Zitnik, Marinka},
journal={Nature Machine Intelligence},
year={2023},
publisher={Nature Springer Group}
}
Code
PyTorch implementation together with documentation and examples of usage is available in the GitHub repository.
Supplementary Information
Review of language model pre-training methods, Supplementary Figures 1–3 and Table 1 are available at Nature Machine Intelligence.