Disease knowledge graphs have emerged as a powerful tool for AI, enabling the connection, organization, and access to diverse information about diseases. However, the relations between disease concepts are often distributed across multiple data formats, including plain language and incomplete disease knowledge graphs. As a result, extracting disease relations from multimodal data sources is crucial for constructing accurate and comprehensive disease knowledge graphs.
We introduce REMAP, a multimodal approach for disease relation extraction. The REMAP machine learning approach jointly embeds a partial, incomplete knowledge graph and a medical language dataset into a compact latent vector space, aligning the multimodal embeddings for optimal disease relation extraction. Additionally, REMAP utilizes a decoupled model structure to enable inference in single-modal data, which can be applied under missing modality scenarios.
We apply the REMAP approach to a disease knowledge graph with 96,913 relations and a text dataset of 1.24 million sentences. On a dataset annotated by human experts, REMAP improves language-based disease relation extraction by 10.0% (accuracy) and 17.2% (F1-score) by fusing disease knowledge graphs with language information. Furthermore, REMAP leverages text information to recommend new relationships in the knowledge graph, outperforming graph-based methods by 8.4% (accuracy) and 10.4% (F1-score).
We introduce REMAP, a multimodal approach for extracting and classifying disease-disease relations. REMAP is a flexible algorithm that can jointly learn over language and graphs and can make predictions even under missing modality scenarios. To achieve this, REMAP specifies graph-based and language-based deep transformation functions that embed each data type separately and optimize unimodal embedding spaces to capture the topology of a disease KG or the text semantics of disease concepts. Finally, to fuse the two data REMAP aligns unimodal embedding spaces a novel alignment penalty loss using disease concepts as anchors. REMAP can effectively data type-specific distribution and diverse representations while aligning embeddings of distinct data types. Furthermore, REMAP can be on both graph and text data types but evaluated and implemented on either of the two modalities alone.
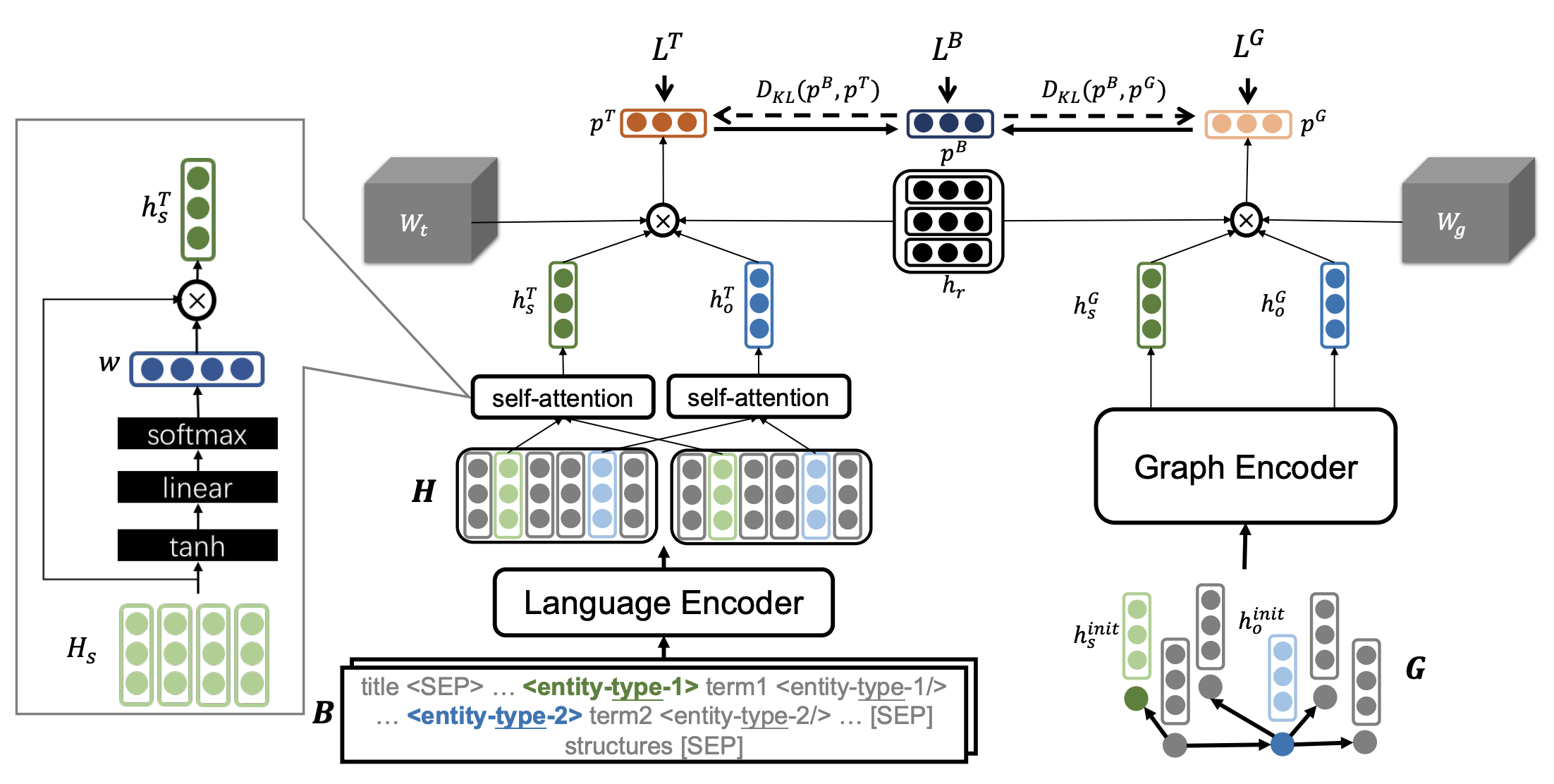
Contributions of this study are:
-
We introduce REMAP, a flexible multimodal approach for extracting and classifying disease-disease relations. REMAP combines knowledge graph embeddings with deep language models and can handle missing data types, which is a critical capability for disease relation extraction.
-
We rigorously evaluate REMAP for finding clinically significant disease-disease relations. We create a training dataset using distant supervision and a high-quality test dataset of gold-standard annotations provided by three clinical domain experts. Our evaluations demonstrate that REMAP achieves an 88.6% micro-accuracy and 81.8% micro-F1 score on the human-annotated dataset, outperforming text-based methods by 10.0% and 17.2%, respectively. Additionally, REMAP achieves the highest performance of 89.8% micro-accuracy and 84.1% micro-F1 score, surpassing graph-based methods by 8.4% and 10.4%, respectively.
Examining the relation between hypogonadism and Goldberg-Maxwell
Hypogonadism and Goldberg-Maxwell syndrome are separate and distinct diseases. However, physicians find it challenging to differentiate in their diagnosis of these two diseases, especially in light of Goldberg-Maxwell syndrome being a rare disease. Differential diagnosis is a process wherein a doctor differentiates between two or more conditions that could be behind a person’s symptoms.
The figure below illustrates how a prediction of “differential diagnosis” (DDx) relationship between hypogonadism and Goldberg-Maxwell syndrome changes from incorrect to a correct prediction when using multimodal learning. REMAP correctly recognizes diseases from text and unifies them with disease concepts in the knowledge graph.
For example, hypogonadism and Goldberg-Maxwell syndrome have many outgoing edges of type differential diagnosis. Further, meta-paths connect these diseases in the graph, such as second-order “differential diagnosis → differential diagnosis” meta-path, and their outgoing degrees in the graph are relatively high. In the case of joint learning, the text-based model can extract part of the disease representation from the graph modality to update its internal representations and thus improve text-based classification of relations.
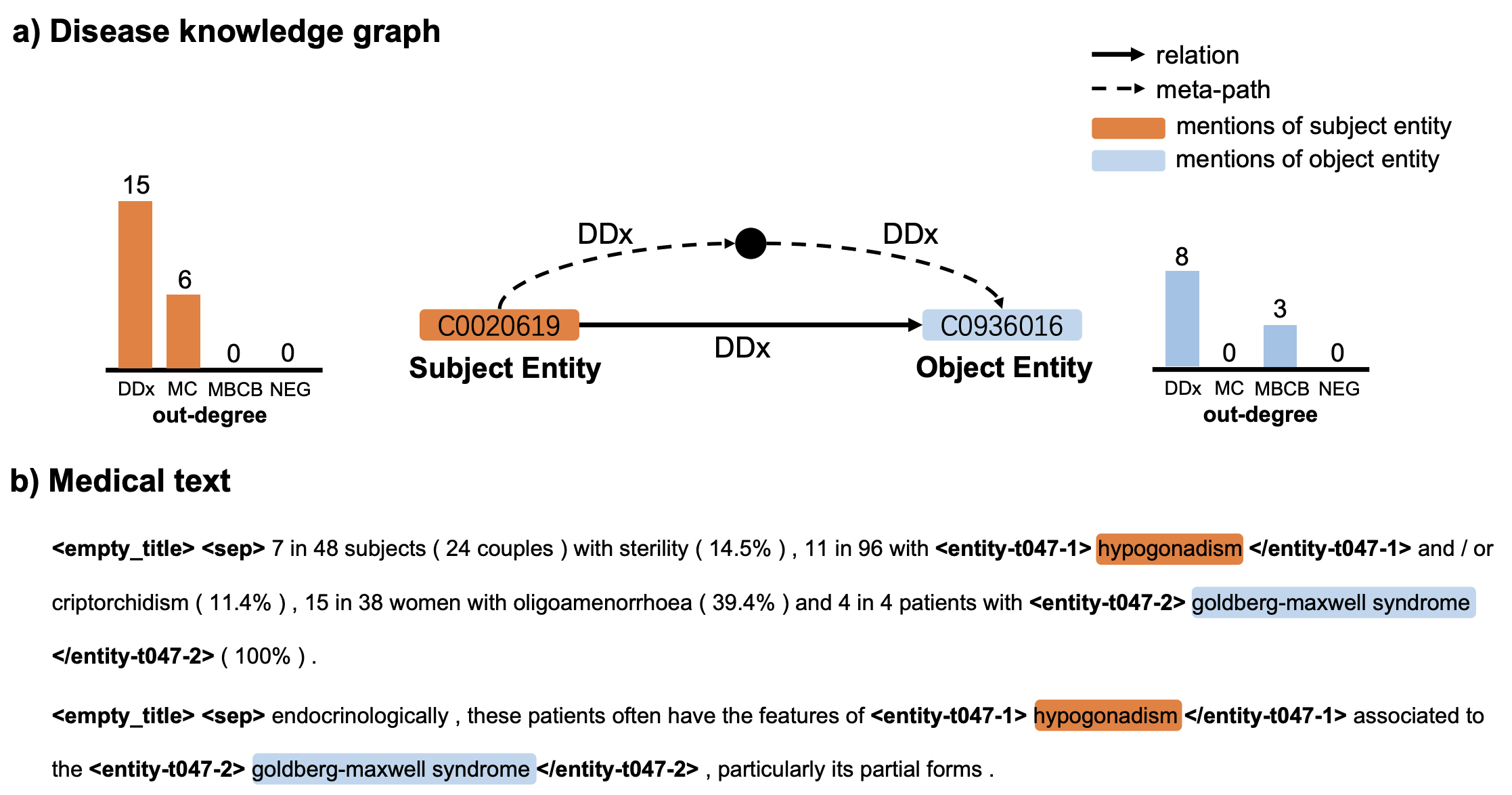
Publication
Multimodal Learning on Graphs for Disease Relation Extraction
Yucong Lin, Keming Lu, Sheng Yu, Tianxi Cai, and Marinka Zitnik
Journal of Biomedical Informatics 2023 [arXiv]
@article{lin2023multimodal,
title={Multimodal Learning on Graphs for Disease Relation Extraction},
author={Lin, Yucong and Lu, Keming and Yu, Sheng and Cai, Tianxi and Zitnik, Marinka},
journal={Journal of Biomedical Informatics},
volume={143},
year={2023}
}
Code
Datasets and Pytorch implementation of REMAP are available in the GitHub repository.