Designing ligand-binding proteins is critical for drug discovery. However, the AI-based design of such proteins is challenging due to the complexity of ligand-protein interactions, the flexibility of ligand molecules and amino acid side chains, and sequence-structure dependencies.
We introduce PocketGen, a deep generative model that simultaneously produces both the residue sequence and atomic structure of the protein regions where ligand interactions occur. PocketGen ensures consistency between sequence and structure by using a graph transformer for structural encoding and a sequence refinement module based on a protein language model. The bilevel graph transformer captures interactions at multiple scales, including atom, residue, and ligand levels. To enhance sequence refinement, PocketGen integrates a structural adapter into the protein language model, ensuring that structure-based predictions align with sequence-based predictions.
PocketGen can generate high-fidelity protein pockets with superior binding affinity and structural validity. It operates ten times faster than physics-based methods and achieves a 95% success rate, defined as the percentage of generated pockets with higher binding affinity than reference pockets. Additionally, it attains an amino acid recovery rate exceeding 64%.
Designing Full-Atom Ligand-Binding Protein Pockets
A primary method for modulating protein functions involves the interaction between proteins and small molecule ligands. These interactions play a critical role in biological processes, such as enzymatic catalysis, signal transduction, and regulatory mechanisms within cells. The binding of small molecules to specific sites on proteins can induce conformational changes, modulate activity, or inhibit functions. This mechanism serves as a valuable tool for studying protein functions and designing small molecule-binding proteins with customized properties for therapeutic and industrial applications, including designing enzymes to catalyze reactions that do not have natural catalysts and developing biosensors that can detect compounds in the environment by transducing signals which can be used for environmental monitoring, clinical diagnostics, pathogen detection, drug delivery systems, and applications in food industry.
These designs often involve modifying existing ligand-binding protein pockets to facilitate more precise interactions with specific ligands. The complexity of ligand molecule-protein interactions, ligand and side chain flexibility, and sequence-structure relationships, however, pose significant challenges for computational generation of high-validity, ligand-binding protein pockets.
Overview of PocketGen
PocketGen implements a co-design scheme, where the model concurrently predicts the sequence and structure of the protein pocket based on the ligand molecule and the protein scaffold (excluding the pocket). PocketGen comprises two modules: the bilevel graph transformer and the sequence refinement module.
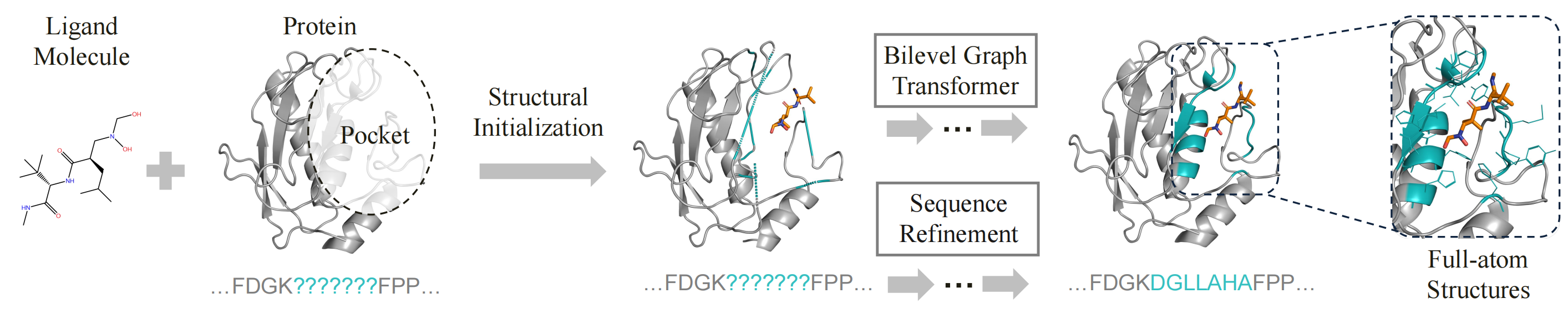
To achieve end-to-end molecular generation, PocketGen models the protein-ligand complex as a geometric graph of blocks to handle variable atom counts across different residues and ligands. Initially, the pocket residues are assigned the maximum possible number of atoms (14 atoms) and later are mapped back to specific residue types post-generation. The bilevel graph transformer captures interactions at multiple granularities (atom-level and residue/ligand-level) and aspects (intra-protein and protein-ligand) using bilevel attention mechanisms. To account for the influence of the redesigned pocket on the ligand, the ligand structure is updated during refinement to reflect potential changes in binding pose.
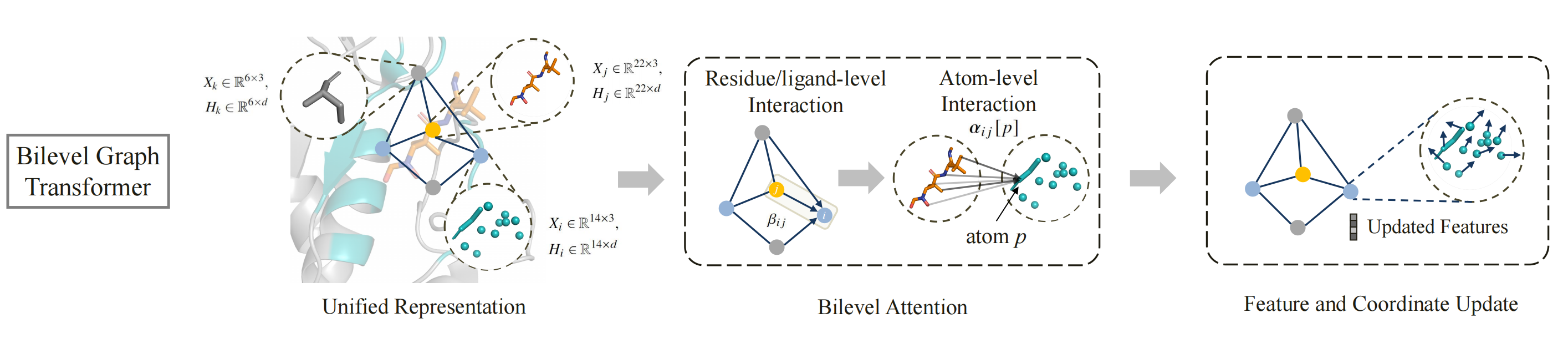
To ensure consistency between protein sequence and structure domains and incorporate evolutionary information encoded in protein language models (the ESM series models), PocketGen implements a structural adapter into protein sequence updates. This adapter facilitates cross-attention between sequence and structure features, promoting information flow and achieving sequence-structure consistency. During training, only the adapter is fine-tuned, while the remaining layers of the protein language models remain unchanged.
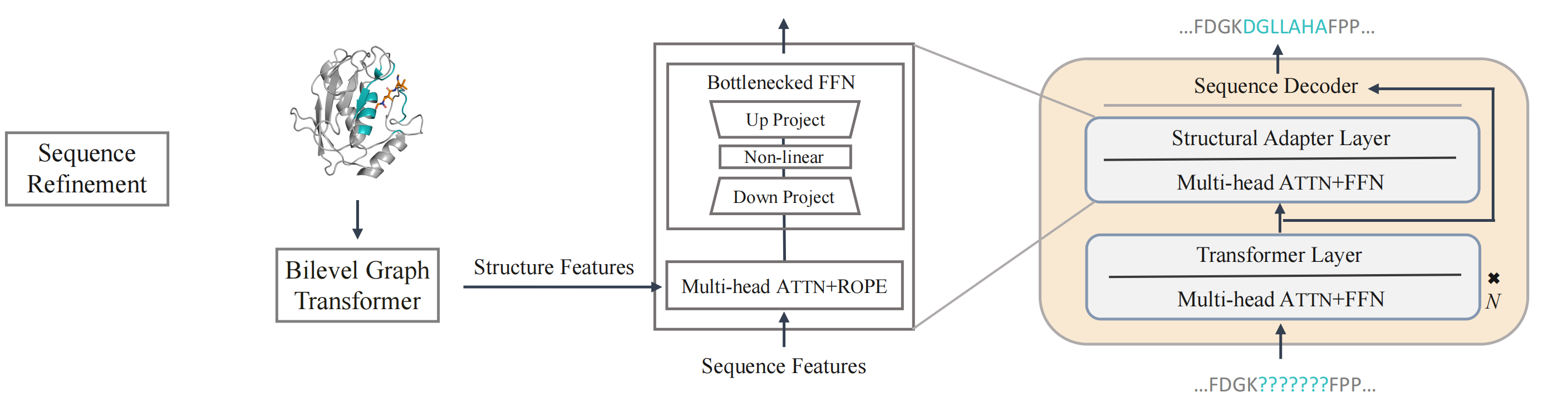
Evaluations of PocketGen
We evaluated PocketGen on redesigning pockets of ligand-binding antibodies, enzymes, and biosensors for the following target ligand molecules:
- Antibodies: Cortisol (HCY) is a primary stress hormone that elevates glucose levels in the bloodstream and serves as a biomarker for stress and other conditions. We redesign the pocket of a cortisol-specific antibody (PDB ID 8cby) to potentially aid in the development of immunoassays.
- Enzymes: Apixaban (APX) is an oral anticoagulant approved by the FDA in 2012 for patients with non-valvular atrial fibrillation to reduce the risk of stroke and blood clots. Apixaban targets Factor Xa (fXa) (PDB ID 2p16), a crucial enzyme in blood coagulation that transforms prothrombin into thrombin for clot formation. Redesigning the pocket of fXa could therefore have therapeutic significance.
- Biosensors: Fentanyl (7V7) has become a widely abused drug contributing to the opioid crisis. Computational design of fentanyl-binding proteins (biosensors) can facilitate detection and neutralization of the toxin. For example, Baker et al. developed a biosensor (PDB ID 5tzo) for detecting fentanyl in plants.
PLIP is employed to describe the interactions between the designed protein pocket and ligands, comparing these predicted interactions to the original patterns. The pockets produced by PocketGen replicate most non-bonded interactions observed in experimentally measured protein-ligand complexes, and introduce additional physically plausible interaction patterns not present in the original complexes.
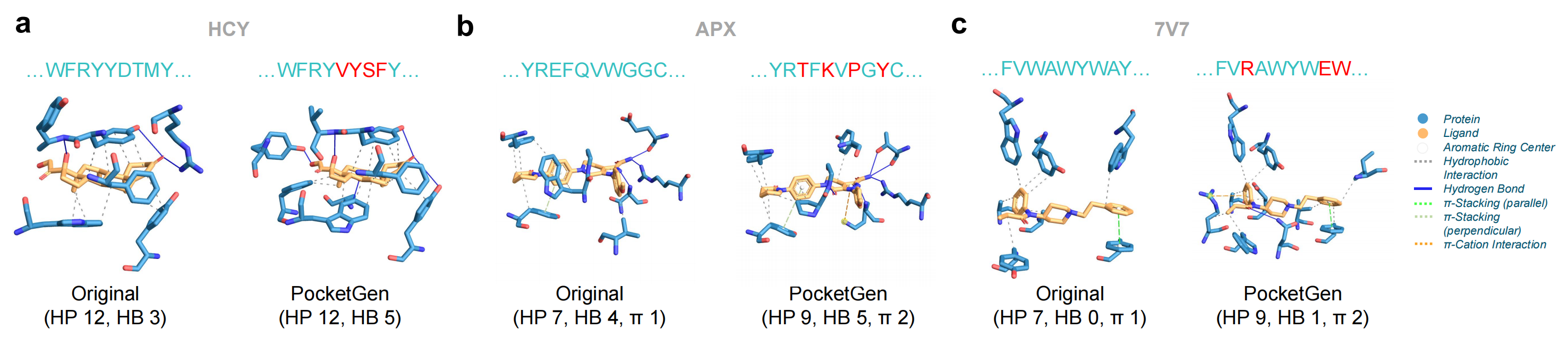
Publication
Efficient Generation of Protein Pockets with PocketGen
Zaixi Zhang, Wanxiang Shen, Qi Liu, Marinka Zitnik
Nature Machine Intelligence 2024 [bioRxiv]
@article{zhang2024pocketgen,
title={Efficient Generation of Protein Pockets with PocketGen},
author={Zhang, Zaixi and Shen, Wanxiang and Liu, Qi and Zitnik, Marinka},
journal={Nature Machine Intelligence},
url={https://www.nature.com/articles/s42256-024-00920-9},
year={2024}
}
Code Availability
Pytorch implementation of PocketGen is available in the GitHub repository.