Designing ligand-binding proteins, such as enzymes and biosensors, is essential in bioengineering and protein biology. One critical step in this process involves designing protein pockets, the protein interface binding with the ligand. Current approaches to pocket generation often suffer from time-intensive physical computations or template-based methods, as well as compromised generation quality due to the overlooking of domain knowledge. To tackle these challenges, we propose PocketFlow, a generative model that incorporates protein-ligand interaction priors based on flow matching. During training, PocketFlow learns to model key types of protein-ligand interactions, such as hydrogen bonds. In the sampling, PocketFlow leverages multi-granularity guidance (overall binding affinity and interaction geometry constraints) to facilitate generating high-affinity and valid pockets. Experiments show that PocketFlow outperforms baselines on multiple benchmarks, e.g., achieving an average improvement of 1.29 in Vina Score and 0.05 in scRMSD. Moreover, modeling interactions make PocketFlow a generalized generative model across multiple ligand modalities, including small molecules, peptides, and RNA.
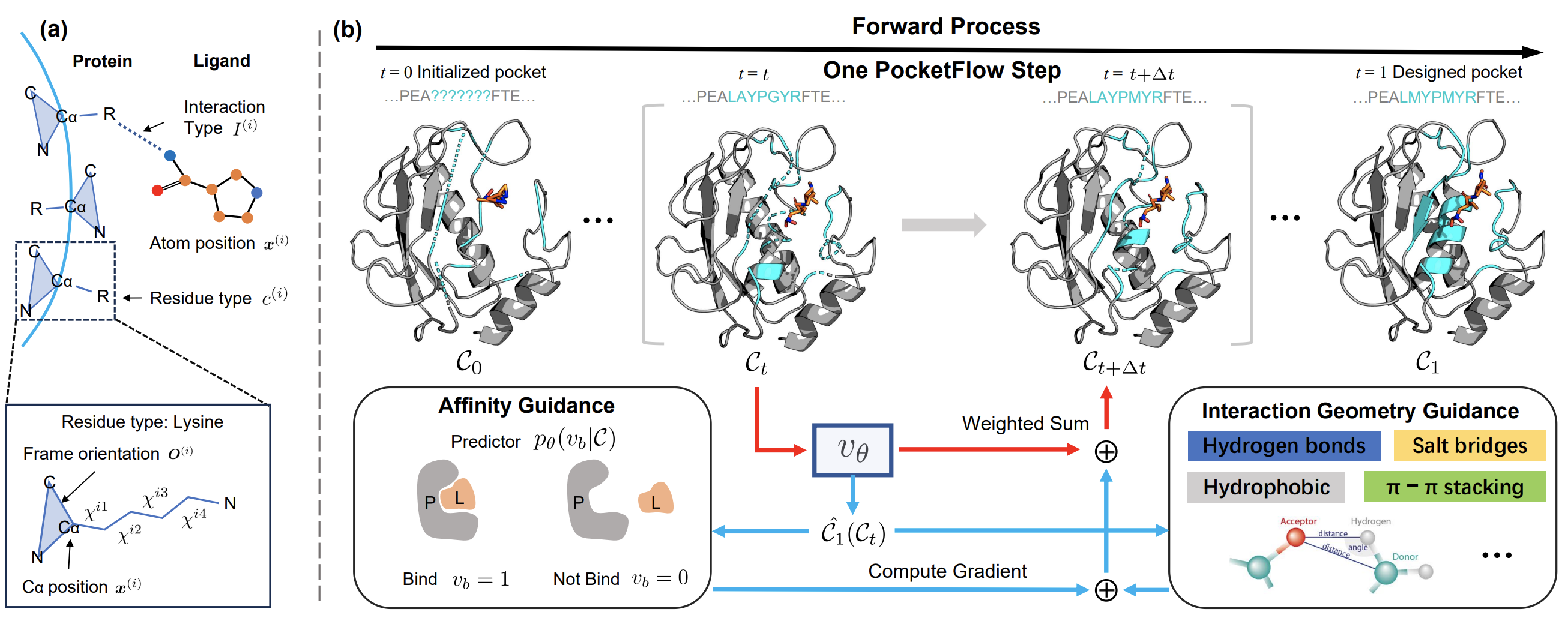
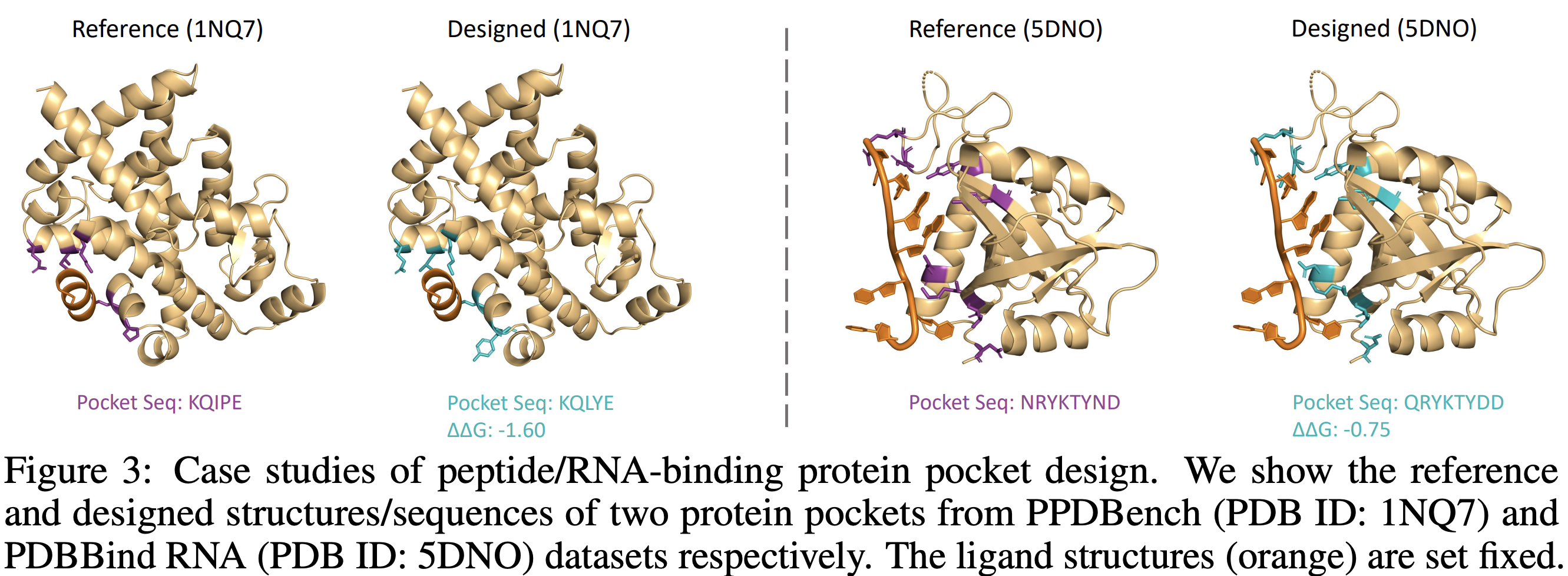
Publication
Generalized Protein Pocket Generation with Prior-Informed Flow Matching
Zaixi Zhang, Marinka Zitnik*, Qi Liu*
NeurIPS 2024 [NeurIPS Spotlight]
@article{zhang2024pocketflow,
title={Generalized Protein Pocket Generation with Prior-Informed Flow Matching},
author={Zhang, Zaixi and Zitnik, Marinka and Liu, Qi},
journal={NeurIPS},
url={https://arxiv.org/abs/2409.19520},
year={2024}
}
Code Availability
Pytorch implementation of PocketFlow is available in the GitHub repository.