As an alternative to target-driven drug discovery, phenotype-driven approaches identify compounds that counteract the overall disease effects by analyzing phenotypic signatures. Our study introduces a novel approach to this field, aiming to expand the search space for new therapeutic agents. We introduce PDGrapher, a causally-inspired graph neural network model designed to predict arbitrary perturbagens – sets of therapeutic targets – capable of reversing disease effects. Unlike existing methods that learn responses to perturbations, PDGrapher solves the inverse problem, which is to infer the perturbagens necessary to achieve a specific response – i.e., directly predicting perturbagens by learning which perturbations elicit a desired response.
Experiments across eight datasets of genetic and chemical perturbations show that PDGrapher successfully predicted effective perturbagens in up to 10% additional test samples and ranked therapeutic targets up to 35% higher than competing methods. A key innovation of PDGrapher is its direct prediction capability, which contrasts with the indirect, computationally intensive models traditionally used in phenotype-driven drug discovery that only predict changes in phenotypes due to perturbations. The direct approach enables PDGrapher to train up to 30 times faster, representing a significant leap in efficiency. Our results suggest that PDGrapher can advance phenotype-driven drug discovery, offering a fast and comprehensive approach to identifying therapeutically useful perturbations.
Target-driven drug discovery, predominant since the 1990s, focuses on the design of highly specific compounds against disease-associated targets such as proteins or enzymes.
- A prime example of target-driven drug discovery is the development of small molecule kinase inhibitors like Imatinib. Imatinib halts the progression of chronic myeloid leukemia (CML) by inhibiting the BCR-ABL tyrosine kinase, a mutated protein that drives uncontrolled proliferation of leukemic cells in CML patients.
- A second notable example are monoclonal antibodies like Trastuzumab which specifically targets the HER2 receptor, a protein overexpressed in certain types of breast cancer. Trastuzumab inhibits cell proliferation while engaging the body’s immune system to initiate an attack against the cancer.
These examples illustrate the success of target-driven approaches, yet the past decade has seen a revival of phenotype-driven approaches. This shift has been fueled by the observation that the majority of the first-in-class drugs approved by the US FDA between 1999 and 2008 were discovered empirically without a drug target hypothesis.
Instead of the one drug, one gene, one disease model of target-driven approaches, phenotype-driven approaches aim to identify compounds, or perturbagens, that reverse phenotypic disease effects as measured by high-throughput screening (HTS) assays. Notable recent successes include Ivacaftor, the first available therapy treating the underlying cause of cystic fibrosis, and Risdiplam, the first oral medicine approved to treat spinal muscular atrophy.
Overview of PDGrapher
Current phenotype-driven lead discovery methods are limited as they identify potential leads by predicting and matching gene expression response signatures with known disease signatures. We propose an alternative approach where the objective is to determine which genes a new perturbagen should target to reverse disease effects as represented by a disease signature. We term this approach combinatorial prediction of therapeutic targets due to its focus on predicting gene combinations.
The problem of finding which elements of a system should be perturbed to achieve a desired outcome is typically referred to as optimal intervention design within the causal discovery literature. Here, we build on this concept to introduce PDGrapher, an approach for the combinatorial prediction of therapeutic targets to shift cell gene expression from an initial to a treated state in genetic and chemical intervention datasets. Our predictive task is formulated using a causal model, where genes are the nodes in a causal graph and structural equations encode their causal relationships. The goal is to identify a set of genes a perturbagen should target to transition nodes states from diseased to treated.
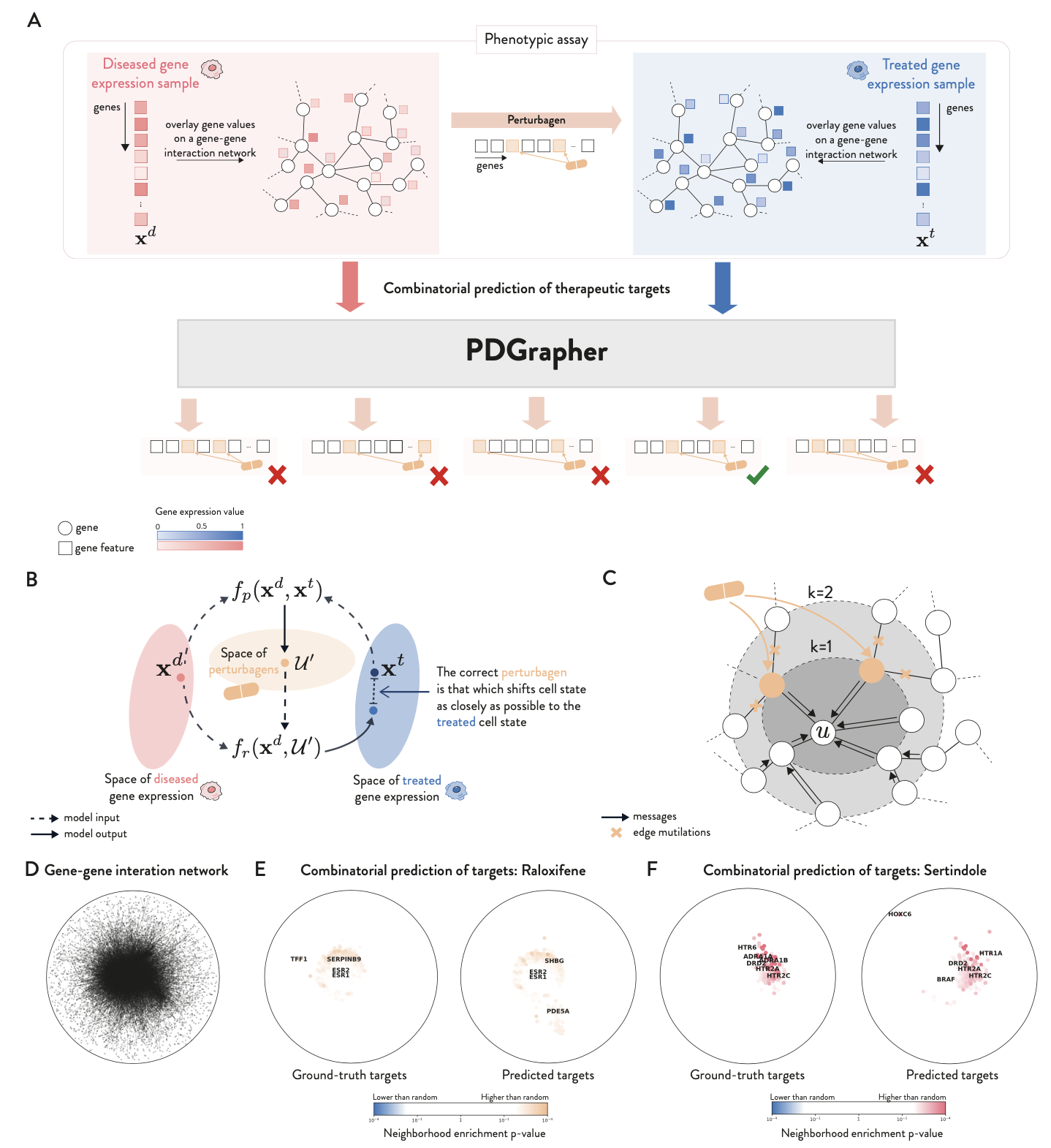
We consider protein-protein interaction networks (PPI) or gene regulatory networks (GRN) as approximations of the causal graph, assuming no unobserved confounders. We tackle our objective through a representation learning approach, using graph neural network (GNN)-based models to implicitly represent the structural equations. For a given pair of diseased and treated samples, PDGrapher outputs a ranking of genes, with the top-predicted genes identified as the primary therapeutic targets that shift gene expression phenotypes from a diseased to a treated state.
We evaluate PDGrapher across four datasets of genetic and chemical interventions in held out folds containing novel samples, and held out folds containing novel samples and cell lines:
- PDGrapher ranks ground-truth therapeutic targets up to 35% higher in chemical intervention datasets and 16% higher in genetic intervention datasets in held out folds containing novel samples, compared with existing approaches.
- In held out folds containing both novel samples and cell lines, PDGrapher maintained its robust performance.
- A key innovation of PDGrapher is its ability to directly predict perturbagens that can shift gene expression from diseased to treated states in contrast with prevailing approaches that indirectly predict perturbagens through extensive computational modeling of cell responses. This enables up to 30 times faster training than existing methods.
- Therapeutic targets predicted by PDGrapher in chemical intervention datasets agree well with ground-truth therapeutic targets, indicating that PDGrapher can elucidate the mode of action for chemical perturbagens and potentially enhance phenotype-driven lead discovery.
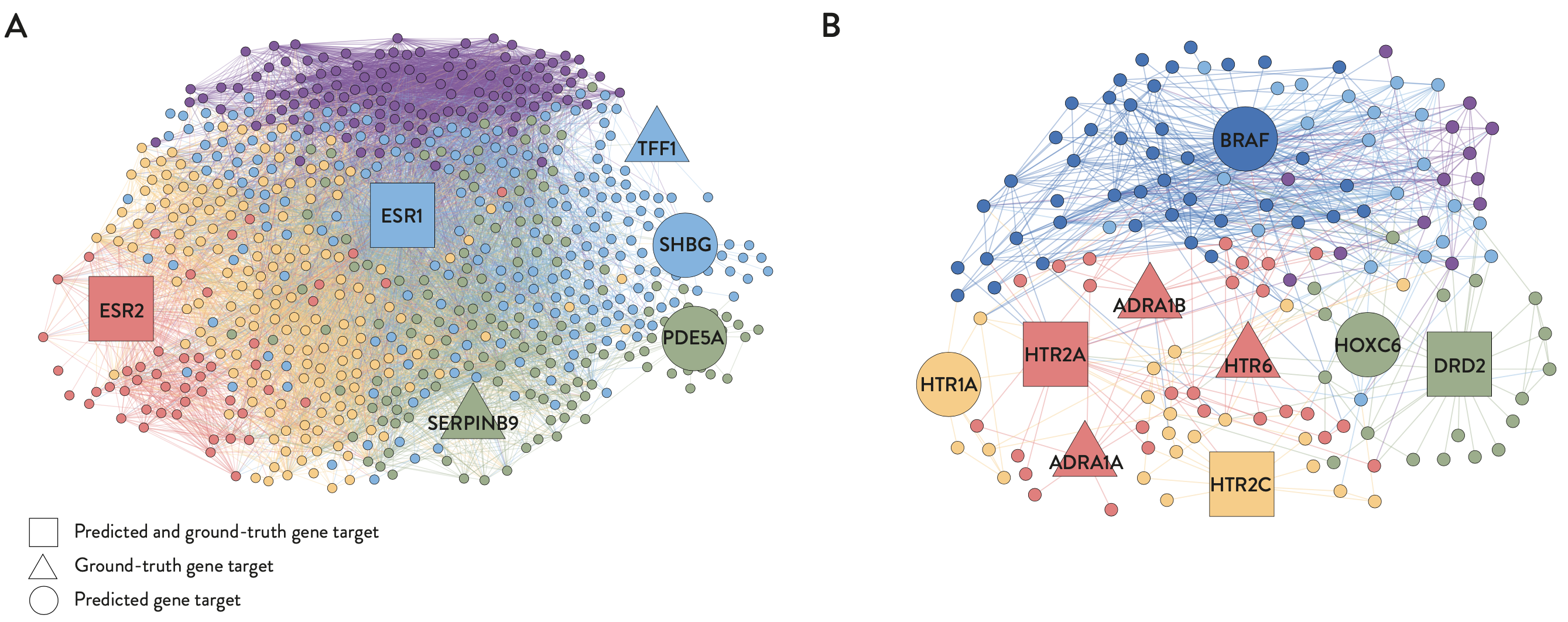
Publication
Combinatorial Prediction of Therapeutic Perturbations Using Causally-Inspired Neural Networks
Guadalupe Gonzalez*, Xiang Lin*, Isuru Herath, Kirill Veselkov, Michael Bronstein and Marinka Zitnik
Nature Biomedical Engineering 2025 [bioRxiv]
@article{gonzalez2025combinatorial,
title={Combinatorial Prediction of Therapeutic Perturbations Using Causally-Inspired Neural Networks},
author={Gonzalez, Guadalupe and Lin, Xiang and Herath, Isuru and Veselkov, Kirill and Bronstein, Michael and Zitnik, Marinka},
journal={Nature Biomedical Engineering},
url={},
year={2025}
}
Code Availability
Pytorch implementation of PDGrapher is available in the GitHub repository.
Data Availability
Processed data, including the cell line gene expression dataset, protein-protein interaction network, drug targets, and disease-associated genes are shared with the research community:
- Protein-protein interaction network data are from BioGrid, Interactome and Interactome Atlas.
- Gene expression datasets are from CLUE.
- Disease-associated genes are from COSMIC and COSMIC Curation.
- Drug target information is extracted from DrugBank.
- List of cancer drugs is obtained from NCI.
Processed dataset is available through Figshare.